Measurement Bias in Statistics and Data Science: Navigating the Pitfalls for Accurate Data Analysis 🧭📊
Hello, data enthusiasts and statistics sleuths! Today, we’re venturing into the intricate world of Measurement Bias in statistics and data science. This journey is crucial because understanding and addressing biases is key to the integrity and usefulness of our data analyses. Let’s unravel the complexities of bias and explore strategies to minimize its impact!
What is Measurement Bias? 🤔⚖️
Measurement Bias refers to any factor that causes a systematic distortion in data collection or analysis. It’s like wearing tinted glasses that skew how you view the world. In the context of data, this bias can significantly affect the accuracy and reliability of your results, leading to misleading conclusions.
The Many Faces of Bias in Data 🎭
- Sampling Bias: Occurs when a sample is not representative of the population. Imagine trying to understand a forest by only looking at one tree! 🌳
- Selection Bias: This happens when the process of selecting participants or data points affects the study’s outcome. It’s like only listening to opinions from people who agree with you. 🙉
- Confirmation Bias: The tendency to search for, interpret, and remember information that confirms pre-existing beliefs. Like a detective only noticing clues that support their theory. 🔍
- Survivorship Bias: Focusing on the survivors or successes while ignoring those that failed or dropped out. It’s like celebrating the few who made it through a tough course while ignoring many who didn’t. 🏆
- Reporting Bias: Arises when certain outcomes are more likely to be reported than others. Think of a news channel that only covers good news. 📰
Minimizing Bias: The Path to Reliable Data 🛤️📈
A. Enhancing Sampling Techniques:
- Random Sampling: Ensure your sample is as random as possible to represent the population accurately.
- Stratified Sampling: Divide the population into strata and randomly sample from each to get a comprehensive view.
B. Refined Research Design:
- Blinding: Keep participants or researchers unaware of certain study aspects to prevent bias.
- Control Groups: Use control groups to compare against the experimental group.
C. Data Collection Vigilance:
- Multiple Data Sources: Use different data sources to cross-check and validate findings.
- Standardized Protocols: Implement and follow standardized procedures for data collection.
D. Analytical Awareness:
- Statistical Adjustments: Use statistical methods to adjust for known biases.
- Peer Review: Encourage peer review of your methods and findings for an unbiased perspective.
E. Ongoing Education and Awareness:
- Bias Awareness Training: Educate yourself and your team on various biases and their impacts.
- Critical Thinking: Cultivate a habit of questioning and critically analyzing your methods and findings.
Conclusion: Steering Clear of Bias for Clearer Insights 🚢🔭
In the vast ocean of data, biases are like hidden currents that can steer your analysis off course. Being aware of these biases and actively working to minimize their influence is essential for any data scientist or statistician. By adopting robust methods and maintaining a critical eye, we can navigate these waters more safely, ensuring that our data analysis is as accurate and reliable as possible.
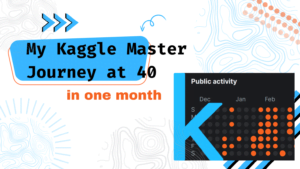