Skewness vs Kurtosis: Deciphering Data Shapes in Statistics 📈🔍
Welcome to the Intricate World of Skewness and Kurtosis in Statistics!
In the diverse landscape of statistical analysis, two important concepts that often intrigue data enthusiasts are skewness and kurtosis. Both play a crucial role in describing the shape and characteristics of data distributions. Let’s delve into these concepts to understand their differences, significance, and applications in the realm of data science. 🚀
What are Skewness and Kurtosis? 🤔
Skewness and kurtosis are measures that describe the shape of a data distribution. While they may sound complex, they are essentially tools to understand how data behaves around the mean.
Skewness: The Asymmetry Measure
- Definition: Skewness measures the degree of asymmetry of a distribution around its mean. It indicates whether the data points are skewed to the left (negative skew) or to the right (positive skew) of the mean.
- Positive Skew: A distribution with a longer tail on the right side.
- Negative Skew: A distribution with a longer tail on the left side.
- Example: Income distribution is often positively skewed, as a majority of people earn below the average, with a few high earners creating a long right tail.
Kurtosis: The Tailedness Measure
- Definition: Kurtosis measures the ‘tailedness’ of a distribution. It describes the height and sharpness of the central peak and the heaviness of the distribution’s tails.
- High Kurtosis (Leptokurtic): A distribution with heavy tails and a sharper peak than a normal distribution.
- Low Kurtosis (Platykurtic): A distribution with lighter tails and a flatter peak.
- Example: A dataset of exam scores where most students performed similarly (high kurtosis) versus a dataset with more varied scores (low kurtosis).
Visualizing Skewness and Kurtosis 📊
Histograms and probability density plots are excellent tools for visualizing skewness and kurtosis. They provide a clear graphical representation of the distribution’s symmetry (or lack thereof) and the prominence of its tails.
The Role of Skewness and Kurtosis in Data Analysis 🔍
- Understanding Data Distribution: Skewness and kurtosis provide insight into the data’s distribution pattern, which is crucial for selecting the right statistical tests and models.
- Identifying Outliers: Skewness, in particular, can help in detecting outliers and understanding their impact on the dataset.
- Predictive Modelling: In machine learning and predictive modeling, knowing the skewness and kurtosis can guide data transformation and normalization processes.
Skewness vs Kurtosis: The Key Differences 🌟
- Focus on Symmetry: Skewness primarily focuses on the symmetry, or lack thereof, of a distribution, whereas kurtosis is more about the extremity of data points.
- Implication on Data Analysis: Skewness affects the direction of data deviation, while kurtosis influences the probability of extreme values.
Let’s include the formulas for both skewness and kurtosis to provide a complete picture:
Skewness Formula
The skewness of a dataset can be calculated using the following formula:
\[ \text{Skewness (G)} = \frac{n}{(n-1)(n-2)} \sum_{i=1}^{n} \left( \frac{x_i – \bar{x}}{s} \right)^3 \]
Where:
- \( n \) is the number of data points in the dataset.
- \( x_i \) represents each individual data point.
- \( \bar{x} \) is the mean of the dataset.
- \( s \) is the standard deviation of the dataset.
This formula calculates the standardized third moment (the sum of the cubed deviations from the mean, divided by the standard deviation cubed), adjusted for bias in small samples.
Kurtosis Formula
Kurtosis is calculated using this formula:
\[ \text{Kurtosis (K)} = \frac{n(n+1)}{(n-1)(n-2)(n-3)} \sum_{i=1}^{n} \left( \frac{x_i – \bar{x}}{s} \right)^4 – \frac{3(n-1)^2}{(n-2)(n-3)} \]
Where the symbols represent the same as in the skewness formula.
This formula calculates the standardized fourth moment (the sum of the fourth power of deviations from the mean, divided by the standard deviation to the fourth power). The term at the end adjusts for the kurtosis of a normal distribution, making the kurtosis of the normal distribution zero (this is sometimes called “excess kurtosis”).
Understanding These Formulas
- Skewness: A skewness value close to 0 indicates a symmetrical distribution. Positive values indicate right skewness, while negative values indicate left skewness.
- Kurtosis: A kurtosis value close to 0 suggests a distribution similar to the normal distribution in terms of its tail’s heaviness. Positive kurtosis indicates heavier tails, while negative kurtosis indicates lighter tails compared to a normal distribution.
Together, these formulas offer a deeper understanding of the distribution’s shape, providing insights that go beyond central tendency and spread.
Conclusion: Embracing the Shapes of Data 🚀
Understanding skewness and kurtosis is like having a deeper conversation with data. It’s about going beyond the averages and medians to explore the subtleties of how data spreads and peaks. As you continue your statistical journey, keep these concepts in mind; they will enrich your understanding of data and enhance your analytical skills.
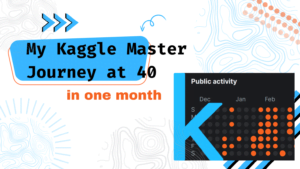