True and Error Scores & Understanding Types of Errors in Statistics: A Data Scientist’s Playbook 📈👩💻
Hello, Data Science Wizards! 🧙♂️🔮 Today, we’re on an adventure through the land of True and Error Scores and exploring the mysterious Types of Errors in statistics. Let’s make this journey engaging and enlightening! 🌟
The Basics: True and Error Scores 🎯
Remember, every score you see is a mix of:
- True Score (T): The real deal, your actual ability or knowledge. 🧠
- Error Score (E): The pesky factors that distort the true score. 🐉
Together, they form:
The Twists: Types of Errors – Random & Systematic 🌪️🔄
In the world of statistics, errors lurk around in two main forms:
Random Errors: Unpredictable, like a roll of dice. 🎲
- Example: Maybe you guessed a couple of answers right on a quiz, boosting your score.
Systematic Errors: Consistent biases, like a scale that’s always off. ⚖️
- Example: A quiz always focusing on topics not covered in lectures.
The Plot Thickens: Type I & Type II Errors 🕵️♂️🕵️♀️
As a data scientist, you’ll often encounter two special types of errors:
Type I Error (False Positive):
- Occurs when you wrongly reject a true null hypothesis.
- Example: Declaring a new drug effective when it’s not. Like a fire alarm going off without a fire. 🔥🚫
Type II Error (False Negative):
- Happens when you fail to reject a false null hypothesis.
- Example: Not detecting a disease in a patient when it’s actually there. Like missing a hidden treasure chest. 💎😓
Why Should Data Scientists Care? 🤔📊
- Better Decision Making: Understanding these errors helps in making more accurate and reliable decisions based on data. 🎲🎯
- Model Accuracy: It’s crucial for evaluating and improving the accuracy of your statistical models. 📈👩🔬
- Risk Management: Helps in assessing and managing the risks associated with false positives and negatives. 🛡️⚠️
- Ethical Implications: Particularly important in fields like medicine or finance, where wrong decisions can have serious consequences. 🏥💸
Final Thoughts: Your Data Science Toolkit 🛠️📚
So, there you go! Understanding True and Error Scores, along with Random, Systematic, Type I, and Type II Errors, is like having a Swiss Army knife in your data science toolkit. These concepts are not just academic; they’re practical tools that help you navigate the complex world of data with more precision and confidence. Embrace them, and you’re on your way to becoming a data science ninja! 🌈🚀
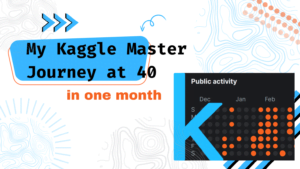