Welcome to the fascinating intersection of traditional sampling methods and the burgeoning world of big data and advanced analytics. In this chapter, we’ll explore how the principles of sampling are more relevant than ever in the era of massive datasets and sophisticated analytical techniques. Let’s navigate through this modern landscape to understand how sampling continues to shape our understanding of data. 🚀📊
5.1 The Continuing Relevance of Sampling in Big Data 🌐
- Big Data vs. Sampling: In an age where data is abundant, sampling remains vital for efficiency, manageability, and cost-effectiveness.
- Real-Life Scenario: Analyzing social media trends – while it’s impractical to analyze every post, a well-chosen sample can provide insightful trends.
5.2 Sampling Techniques for Large Datasets: Adapting Traditional Methods 🔄
- Random Sampling in Big Data: Ensuring representativeness in vast datasets.
- Stratified Sampling in Diverse Data: Handling large datasets with varied subgroups to maintain accuracy and diversity.
- Example in E-commerce: Using stratified sampling to analyze customer behavior across different product categories.
5.3 The Role of Sampling in Machine Learning and AI 🤖
- Training Machine Learning Models: Using samples to train algorithms efficiently and effectively.
- Challenges in Sampling for AI: Ensuring that the training data is representative to avoid biases in machine learning models.
5.4 Quality Over Quantity: Why More Data Isn’t Always Better 📉
- The Pitfalls of Excessive Data: Overwhelming amounts of data can lead to noise and confusion.
- The Power of Well-Chosen Samples: Extracting meaningful insights from well-curated subsets.
5.5 Sampling in Qualitative vs. Quantitative Research: Different Needs, Different Approaches 🔍
- Quantitative Sampling: Focused on numerical analysis, often using random or stratified techniques.
- Qualitative Sampling: More about depth than breadth, often using purposive or snowball sampling methods.
- Balancing Both Worlds: A case study in market research combining quantitative and qualitative approaches.
5.6 Ethical and Privacy Considerations in Sampling: Navigating the Digital Age 🛡️
- Data Privacy in Sampling: Addressing concerns in an era of heightened awareness about personal data.
- Ethical Sampling Practices: Ensuring that data collection and sampling respect privacy and consent, particularly in sensitive areas like healthcare or personal finance.
5.7 Advanced Sampling Techniques: The Future Is Now 🚀
- Predictive Sampling: Leveraging AI to predict and model future trends.
- Responsive and Adaptive Sampling: Techniques that evolve in real-time with data changes.
Conclusion: Sampling – The Timeless Navigator of the Data Sea 🌟
In the vast sea of data that characterizes our modern world, sampling remains an indispensable navigator. It guides us to meaningful insights, ensuring that our journey through the waves of big data and advanced analytics is both efficient and effective. As we embrace the future, the principles of sampling stand as a testament to the enduring value of this fundamental statistical practice.
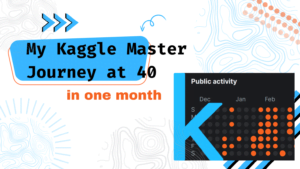