In the journey through the statistical landscape, advanced sampling techniques represent the frontier of innovation and adaptability. This chapter delves into sophisticated methods designed to meet the unique challenges posed by diverse and dynamic data environments. Let’s explore these advanced techniques, understanding how they enhance our capacity to draw meaningful insights from complex data sets. 🌐🚀
6.1 Multistage and Multiphase Sampling: Complexity Simplified 🌟
- Multistage Sampling: This technique involves multiple levels of sampling and is often used in large-scale surveys. It’s like zooming in from a bird’s-eye view to a street-level perspective in stages.
- Example in Practice: National health surveys where regions are first sampled, then specific communities, and finally households.
- Multiphase Sampling: Here, different methods or phases of sampling are combined for comprehensive data collection.
- Application Scenario: In ecological studies, initially surveying areas via remote sensing and subsequently conducting ground-level biological surveys.
6.2 Adaptive Sampling: The Responsive Approach 🔄
- The Concept: Adaptive sampling adjusts the sampling strategy based on initial findings. It’s like altering your route based on the terrain you encounter.
- Real-World Example: Wildlife researchers might increase sampling density in areas where they initially find more signs of a rare species.
6.3 Sampling in Time-Series and Panel Data: Capturing Change Over Time ⏳
- Time-Series Sampling: This method involves collecting data at regular intervals to analyze trends over time.
- Example: Financial analysts sampling stock prices at regular intervals to identify market trends.
- Panel Sampling: Sampling the same subjects at different times to observe changes.
- Application: Longitudinal studies in social science where the same group of individuals is surveyed over several years.
6.4 High-Dimensional Data Sampling: The Big Data Challenge 📊
- Navigating Large Dimensions: In datasets with numerous variables, traditional sampling techniques may fall short. High-dimensional sampling methods are tailored to handle this complexity.
- Use Case: Genomic studies where researchers deal with thousands of genetic markers.
6.5 The Role of AI and Machine Learning in Sampling: A Tech-Driven Future 🤖
- AI-Enhanced Sampling: Leveraging artificial intelligence to identify patterns and guide the sampling process.
- Machine Learning Applications: Using predictive models to determine optimal sampling strategies for complex datasets.
6.6 The Intersection of Sampling and Data Mining: Unearthing Hidden Gems 💎
- Data Mining Sampling: Sampling methods that are specifically designed for data mining processes, helping to extract actionable insights from large datasets.
- Application in Retail: Analyzing customer transaction data to identify purchasing patterns and optimize inventory management.
6.7 Ethical Sampling in a Digital Age: Responsibility and Challenges 🛡️
- Ethical Dilemmas: Addressing concerns around bias, privacy, and consent in advanced sampling methods.
- Balancing Innovation with Integrity: Ensuring that new sampling techniques adhere to ethical standards and respect individual rights.
Conclusion: Advanced Sampling – Navigating the Future of Data Analysis 🚀
Advanced sampling techniques represent the cutting edge of statistical methodology, offering innovative solutions to modern data challenges. As data continues to grow in volume, variety, and velocity, these advanced methods will play a pivotal role in shaping the future of data analysis, offering new horizons of understanding and insight.
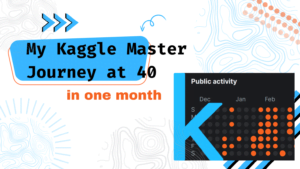
Chapter 8: The Future of Sampling in Statistics – Emerging Trends and Innovations
November 30, 2023
5 Comments
Read More »
Facebook
Twitter
LinkedIn
Hi, Welcome back!