Population Mean vs. Sample Mean: Navigating the World of Averages 🌐📊
Welcome to the Intriguing World of Means!
In the realm of statistics and data science, two heroes emerge when we talk about averages: the Population Mean and the Sample Mean. While they might sound similar, they play distinct roles in how we interpret data. Understanding the difference between these two is not just a statistical necessity; it’s the key to accurate data interpretation and analysis. Let’s explore these concepts in detail. 🚀
Population Mean: The Big Picture 🌍
The Population Mean (μ) is like an aerial view of a landscape, providing a comprehensive picture. It represents the average of all values in a population – the entire group you’re interested in studying.
- Definition: The Population Mean is the sum of all the values in the population divided by the number of values in that population.
- Equation: \[ \mu = \frac{\sum_{i=1}^{N} X_i}{N} \]
Here, \( \mu \) is the population mean, \( X_i \) represents each value in the population, and \( N \) is the total number of values. - Use Case: Ideal for smaller, well-defined populations or when you have access to complete data.
- Example: The average age of all the students in a small school.
Sample Mean: The Practical Approach 📈
In contrast, the Sample Mean ( \( \bar{x} \) ) is like zooming in on a specific part of the landscape. It deals with a subset of the population, providing an estimate of the population mean.
- Definition: The Sample Mean is the average of all values in a sample, which is a representative segment of the population.
- Equation:\[ \bar{x} = \frac{\sum_{i=1}^{n} x_i}{n} \]
Here, \( \bar{x} \) is the population mean, \( x_i \) represents each value in the population, and \( n \) is the total number of values. - Use Case: Used when it’s impractical or impossible to examine the entire population.
- Example: The average age of 50 randomly selected students from the same school.
Key Differences: Understanding the Nuances 🚧
- Scope and Accessibility: The Population Mean encompasses the whole group, while the Sample Mean focuses on a part of that group.
- Purpose and Application: The Population Mean provides the true average, while the Sample Mean is a practical estimation, often used in inferential statistics to draw conclusions about the population.
- Representation: They are represented differently in equations, with μ for the population mean and \( \bar{x} \) for the sample mean.
Why Both are Important? 🌟
- Feasibility and Practicality: In many real-world scenarios, especially with large populations, it’s only feasible to calculate the sample mean.
- Inference and Prediction: The sample mean allows statisticians and data scientists to make predictions about the population mean, which is especially useful in surveys and experiments.
Conclusion: Balancing the Big Picture with Practical Insights 🎯
Understanding the difference between the population mean and the sample mean is crucial in statistics. It’s about balancing the comprehensive view provided by the population mean with the practical insights offered by the sample mean. Whether you are a researcher, a data scientist, or a student, recognizing when to use each will enhance the accuracy and reliability of your data analysis.
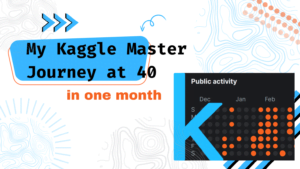