Cross validation is an important technique used in machine learning model evaluation and selection. Here’s a brief overview:
It is used to evaluate how the results of a statistical model will generalize to an independent dataset.
The dataset is divided into k number of groups known as folds. Typically k=5 or 10.
One fold is used as the validation set to evaluate the model, while the remaining k-1 folds are used to train the model.
This process is repeated k times, each time using a different fold as the validation set.
The validation results are then averaged over all k trials to get an overall cross-validation estimate of how the model is expected to perform.
This helps address overfitting – models that perform well only due to a particular dataset split.
Common types include k-fold CV, leave-one-out CV, stratified CV etc. depending on the problem.
It provides an almost unbiased estimation of model performance on unseen data without a separate hold-out test set.
Popular in model selection to choose hyperparameters that generalize better to new examples.
So in summary, cross validation helps address overfitting and identify how well a model can classify or predict unknown examples. It is a standard evaluation technique in ML.
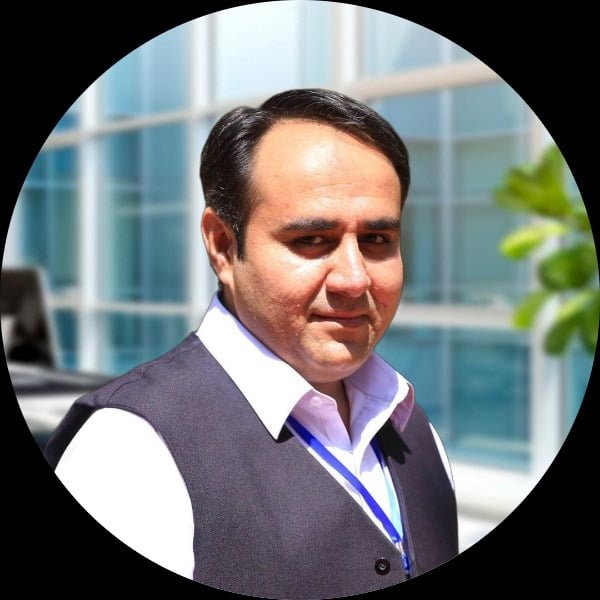