About Lesson
Here are some common types of activation functions used in TensorFlow:
💪 Rectified Linear Unit (ReLU): f(x) = max(0, x)
- Most widely used activation function. Works well for both shallow and deep networks.
🙂 Sigmoid: f(x) = 1/(1+e-x)
- Squashes the output to range between 0-1. Used for probability predictions in the output layer.
🤙 Tanh (Hyperbolic Tangent): f(x) = (e^x – e^-x)/(e^x + e^-x)
- Squashes output to range -1 to 1. ReLU often works better than tanh in hidden layers.
🤓 Softmax: f(x)_i = e^{x_i} / ∑_j e^{x_j}
- Used for multi-class classification where the outputs represent class probabilities.
😎 Leaky ReLU: f(x) = max(αx, x) where α is a small positive value like 0.01
- Solves the “dying ReLU” problem where a unit may stop learning if its input is negative.
😎 ELU (Exponential Linear Unit): f(x) = x for x > 0, f(x) = α(e^x – 1) for x < 0
- Slightly better than ReLU by allowing negative values close to zero.
So in summary, ReLU, sigmoid, tanh are commonly used in hidden layers while softmax is popular in output layers for classification tasks.
Join the conversation
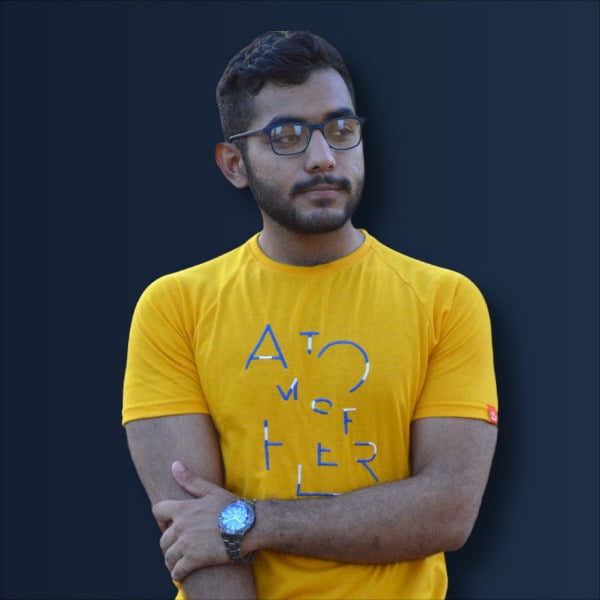
Types of Activation Functions that I know of so far in life:
Sigmoid Activation Function: 1/(1+e^-x)
Tanh Activation Function: (e^x - e^-x)/(e^x + e^-x)
Relu Activation Funtion: max(0,x)
Leaky Relu Activation Function: max(0.1x,x)
Parametric Relu Activation Function: max(ax,x)
Softmax Activation Function: e(i) / sum of e(i...)
Reply
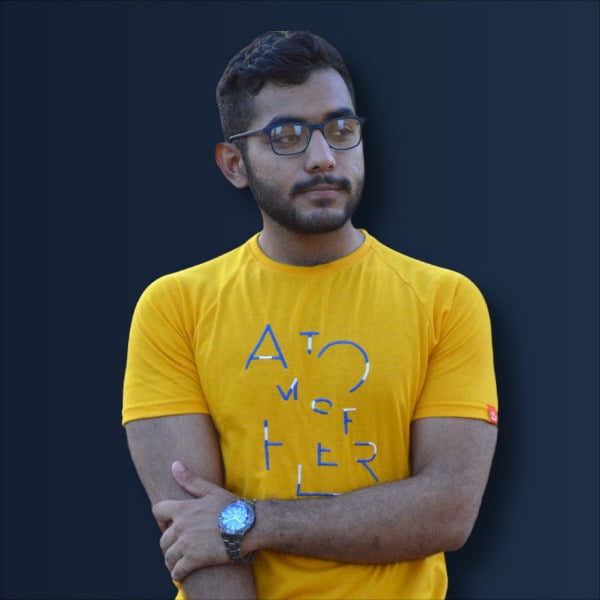
Output Layer ...
Reply
output layer
Reply