Data Distribution in Data Science: Navigating Through the World of Statistical Patterns ๐๐
Welcome to the Enthralling World of Data Distribution in Data Science!
In the vast and ever-evolving field of data science, understanding data distributions is akin to mastering the language of data. These distributions provide crucial insights into the nature, behavior, and patterns inherent in datasets, guiding data scientists in their quest to uncover meaningful insights. Let’s embark on an exploratory journey through the various types of data distributions and their significance in the realm of data science. ๐
What is Data Distribution? ๐ค
Data distribution refers to how data points in a dataset are spread out or clustered across a range of values. It is the blueprint that describes the shape or spread of data in a graphical format. Understanding the distribution of data is fundamental in choosing the correct statistical methods and models for analysis.
Key Types of Data Distributions: A Spectrum of Patterns ๐
Data distributions come in various shapes and forms, each with unique characteristics and implications:
1. Normal Distribution: The Bell Curve
- Characteristics: Symmetrical, bell-shaped curve centered around the mean.
- Significance: Often considered the default pattern in many statistical analyses due to its predictability and the central limit theorem.
- Example: Human heights within a specific gender and age group.
2. Uniform Distribution: Even Spread
- Characteristics: All values have the same frequency, creating a flat distribution.
- Example: A fair roll of a dice, where each outcome from 1 to 6 is equally likely.
3. Binomial Distribution: Success or Failure
- Characteristics: Represents the probability of a fixed number of successes in a series of independent experiments.
- Example: The number of heads observed when flipping a coin multiple times.
4. Poisson Distribution: Counting Events
- Characteristics: Models the number of times an event occurs within a fixed interval.
- Example: The number of emails a person receives per day.
5. Exponential and Gamma Distributions: Modeling Time
- Characteristics: Often used to model waiting times or lifetimes.
- Example: The amount of time until the next bus arrives.
Visualizing Data Distributions: The Power of Plots ๐
Graphical representations such as histograms, box plots, and probability density plots are invaluable in visualizing data distributions. They transform complex numerical concepts into easily interpretable visual formats, facilitating better understanding and decision-making.
The Role of Data Distribution in Data Science ๐
Understanding the distribution of data is pivotal in data science for several reasons:
- Model Selection: Different distributions require different statistical models and methods.
- Predictive Analysis: Distributions help in making accurate predictions and estimations.
- Outlier Detection: Certain distributions assist in identifying and interpreting outliers in data.
Navigating Through Distributions: The Path to Insightful Data Analysis ๐
Grasping the concept of data distribution equips data scientists with the knowledge to make informed choices about data analysis techniques, ensuring accurate and meaningful results. It’s the compass that guides them through the complex world of data.
Conclusion: Embracing the Diversity of Data Distributions ๐
In conclusion, data distributions are at the heart of data science. They offer a window into the soul of datasets, revealing patterns, tendencies, and characteristics crucial for insightful analysis. As you venture further into data science, let the understanding of data distributions be your guiding star in the vast universe of data analysis.
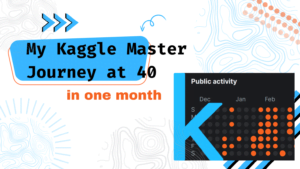
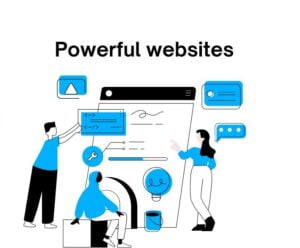
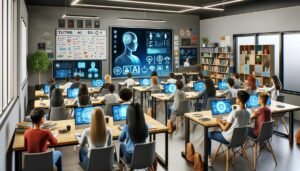
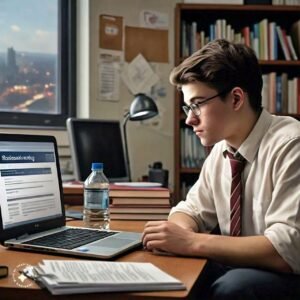
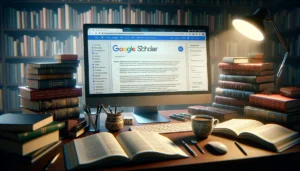
This blog tells us the importance of distribution in the context of data normalization.
nice bht aala
AOA, This blog provides a comprehensive and insightful explanation of different types of data distributions and their significance in data science. It effectively conveys the importance of understanding data distribution for model selection, predictive analysis, and outlier detection. The inclusion of visualizations and real-life examples enhances the understanding of the concepts. It is a valuable resource for me in terms of data distribution and statistics. ALLAH PAK ap ko dono jahan ki bhalian aata kry AAMEEN.
Nicely defined the concepts of Data Distributions in statistics … Easy to understand this blog.
Thank u sir