True and Error Scores & Understanding Types of Errors in Statistics: A Data Scientist’s Playbook 📈👩💻
Hello, Data Science Wizards! 🧙♂️🔮 Today, we’re on an adventure through the land of True and Error Scores and exploring the mysterious Types of Errors in statistics. Let’s make this journey engaging and enlightening! 🌟
The Basics: True and Error Scores 🎯
Remember, every score you see is a mix of:
- True Score (T): The real deal, your actual ability or knowledge. 🧠
- Error Score (E): The pesky factors that distort the true score. 🐉
Together, they form:
The Twists: Types of Errors – Random & Systematic 🌪️🔄
In the world of statistics, errors lurk around in two main forms:
Random Errors: Unpredictable, like a roll of dice. 🎲
- Example: Maybe you guessed a couple of answers right on a quiz, boosting your score.
Systematic Errors: Consistent biases, like a scale that’s always off. ⚖️
- Example: A quiz always focusing on topics not covered in lectures.
The Plot Thickens: Type I & Type II Errors 🕵️♂️🕵️♀️
As a data scientist, you’ll often encounter two special types of errors:
Type I Error (False Positive):
- Occurs when you wrongly reject a true null hypothesis.
- Example: Declaring a new drug effective when it’s not. Like a fire alarm going off without a fire. 🔥🚫
Type II Error (False Negative):
- Happens when you fail to reject a false null hypothesis.
- Example: Not detecting a disease in a patient when it’s actually there. Like missing a hidden treasure chest. 💎😓
Why Should Data Scientists Care? 🤔📊
- Better Decision Making: Understanding these errors helps in making more accurate and reliable decisions based on data. 🎲🎯
- Model Accuracy: It’s crucial for evaluating and improving the accuracy of your statistical models. 📈👩🔬
- Risk Management: Helps in assessing and managing the risks associated with false positives and negatives. 🛡️⚠️
- Ethical Implications: Particularly important in fields like medicine or finance, where wrong decisions can have serious consequences. 🏥💸
Final Thoughts: Your Data Science Toolkit 🛠️📚
So, there you go! Understanding True and Error Scores, along with Random, Systematic, Type I, and Type II Errors, is like having a Swiss Army knife in your data science toolkit. These concepts are not just academic; they’re practical tools that help you navigate the complex world of data with more precision and confidence. Embrace them, and you’re on your way to becoming a data science ninja! 🌈🚀
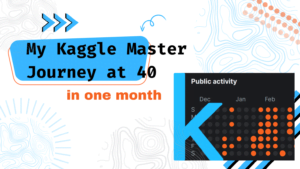
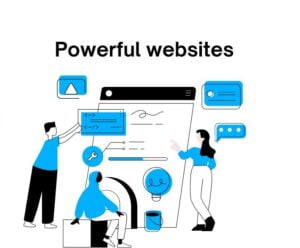
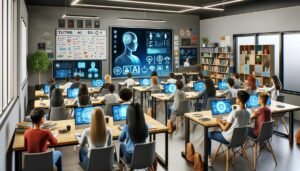
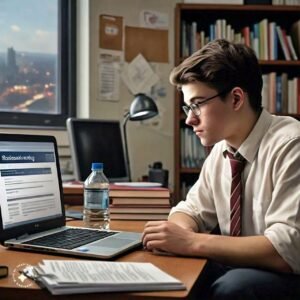
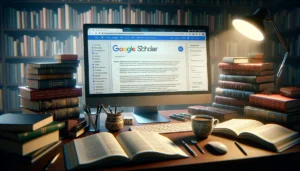
nice
Explanation of true and error scores, types of errors, and type-I and type-II errors is very helpful. In my understanding, we can minimize error by applying the WH question to data.
AOA, I found this blog to be a comprehensive and insightful explanation of the concept. The blog effectively introduces the concepts of true scores and error scores, emphasizing their significance in statistical analysis. It also discusses the different types of errors, including random and systematic errors, as well as Type I and Type II errors. The blog post provides practical examples and highlights the importance of understanding and addressing these errors in data analysis and decision-making. It is a valuable read that enhanced my understanding of true and error scores in statistics. ALLAH PAK ap ko dono jahan ki bhalian aata kry AAMEEN.
Nicely Explained of this topic True and Error Scores …
Respected Sir!
you deliver Completely clear concept about random and systematic erros and thier types
well explained blog on True and Error score and Types of Error and it helps alot to understand the need of resolving these errors