Triangulation in Research: Navigating the Maze of Data for Accurate Insights 🌐🔍
Welcome, curious minds and data enthusiasts! Today, we’re embarking on an exploratory journey into the world of Triangulation in research. This isn’t just a term for geographers or sailors; it’s a powerful tool in the arsenal of modern researchers, particularly in data science. So, grab your compasses and let’s navigate through the maze of data using the art and science of Triangulation!
What is Triangulation? 🤔📐
Triangulation, in the broadest sense, is like using multiple maps and landmarks to pinpoint your exact location. In research, it involves combining multiple data sources, methods, or theories to cross-check and validate findings. It’s a way to ensure that the conclusions we draw are not just a one-off anomaly but a consistent pattern observed from different angles.
The Pillars of Triangulation 🏛️
- Data Triangulation: Gathering data from different sources to answer the same question.
- Methodological Triangulation: Using different methods or techniques to collect and analyze data.
- Theoretical Triangulation: Applying different theories or perspectives to interpret the data.
- Investigator Triangulation: Involving multiple researchers or observers in the study.
Real-World Examples: Triangulation in Action 🌍
Studying Urban Pollution 🏙️🔬
Researchers might combine direct air quality measurements, health records of residents, and satellite imagery to understand the impact of pollution on a city. This approach ensures that the findings are not skewed by anomalies in any single data source or method.
Market Research in Business 🛍️💼
A company might use customer surveys, sales data, and social media sentiment analysis to gauge consumer preferences. This triangulation helps in making robust marketing strategies that resonate with diverse customer insights.
Historical Research 📜🕰️
Historians often use triangulation by combining primary sources (like letters, diaries), secondary sources (like textbooks, scholarly articles), and archaeological evidence to construct accurate historical narratives.
Why Triangulation Matters in Research 🌟
- Enhances Credibility: By using multiple lenses to view data, the research becomes more credible and well-rounded.
- Reduces Bias: It helps in mitigating the bias that might arise from a single method, source, or theory.
- Increases Confidence in Findings: Researchers can be more confident about their conclusions when different methods converge on the same result.
- Offers a Comprehensive View: Triangulation allows for a more holistic understanding of complex phenomena.
Challenges and Considerations 🚧
- Resource Intensive: Triangulation can be time-consuming and may require more resources.
- Complexity in Integration: Integrating findings from different methods or theories can be challenging.
- Skill Requirements: Researchers need to be skilled in multiple methodologies and theories.
Conclusion: The Compass of Modern Research 🧭
Triangulation is not just a fancy term in research; it’s an essential approach in the quest for truth and accuracy in the age of information overload. By employing this method, researchers can navigate through the complexities of data and draw insights that are not just accurate but also holistic. Whether it’s in the realms of social sciences, business, or even natural sciences, triangulation serves as the compass that guides researchers towards more reliable and comprehensive understanding.
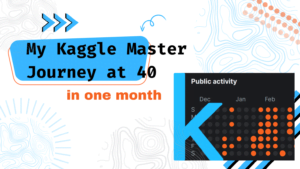
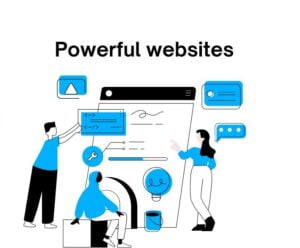
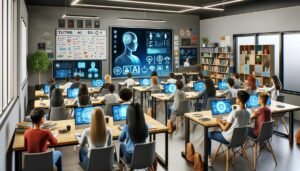
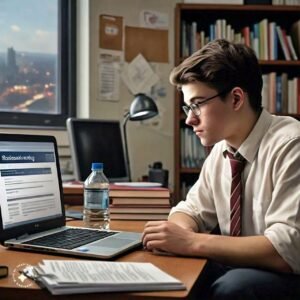
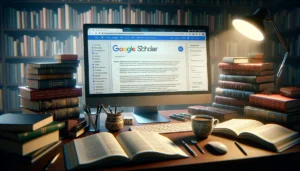
Triangulation means the result for data remains the same when we research it through three different methods. Moreover, triangulation Implementation is also beneficial for data validity.
Ok
AOA, This statistics blog on “Triangulation in Research” is an insightful and informative piece. It effectively explains the concept of triangulation and its significance in research, particularly in the field of data science. This blog discusses the various types of triangulation, including data triangulation, methodological triangulation, theoretical triangulation, and investigator triangulation, providing clear examples for each. It also highlights the benefits of triangulation, such as enhancing credibility, reducing bias, increasing confidence in findings, and offering a comprehensive view of complex phenomena. Additionally, this blog acknowledges the challenges and considerations associated with triangulation. It is a well-written and valuable resource for researchers seeking a deeper understanding of triangulation in research. ALLAH PAK ap ko dono jahan ki bhalian aata kry AAMEEN.
Such an amazing way to describe the concept of Triangulation in Research
Triangulation k under hm apna koi bh research ka result leny k ly diffent methods use karen but hmari research k relevent hu ya phr apni ksi bh problem ko solve krny k ly different methods use karen jo hamen us k related solution provide karen
This content, it’s very useful for increase the knowledge of data science, and enhance my confidence when i reading this kind of blogs. Thank you #babaG
Triangulation: if we wanna check any result with multiple methods and get same results it may called triangulation. E.g if a store get drip in sales of particular item . 1. It can be checked by customer reviews. 2. Recheck with other stores sales. 3. Recheck with manufacturer that they have get any drop in sales.
Example population vs sample:::
conducting a survey to find out how many people in a city prefer a particular brand of coffee. If we survey every resident, that’s the population. On the other hand, if we randomly select 100 residents and survey them, that’s your sample.