Join the conversation
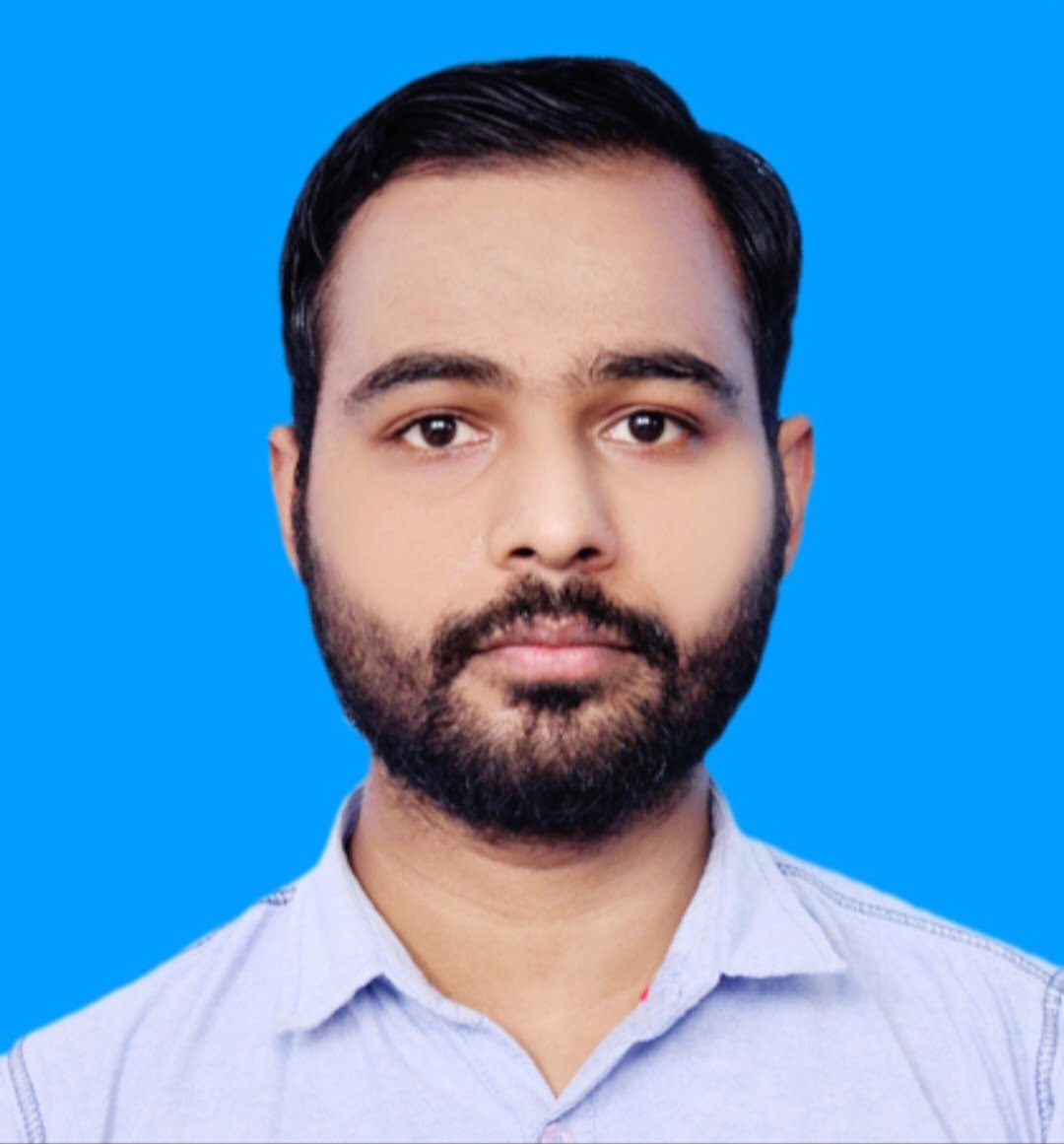
Done
Reply

1. Global Outliers (Point Outliers)
Definition: A data point that deviates significantly from the rest of the dataset.
Example: In a dataset of student ages (10-15 years), an entry with age 50 is a global outlier.
2. Contextual Outliers (Conditional Outliers)
Definition: A data point that is only an outlier in a specific context.
Example: A temperature of 30°C is normal in summer but an outlier in winter.
3. Collective Outliers
Definition: A group of data points that, when considered together, show abnormal behavior, but individually they may not be outliers.
Example: In a network traffic dataset, a sudden spike in traffic from multiple IPs at the same time could indicate a DDoS attack.
4. Natural Outliers
Definition: Outliers that are naturally occurring and not errors.
Example: In a sports dataset, Usain Bolt's 100m sprint time is much lower than others, but it's a natural outlier due to his exceptional performance.
5. Human-made Outliers (Error-based Outliers)
Definition: Outliers caused by data entry errors or sensor malfunctions.
Example: A patient's height recorded as 300 cm instead of 180 cm due to a typo.
1. Univariate Outliers
Definition: Outliers detected by analyzing a single feature (variable) independently.
Example:
In a dataset of salaries, if most salaries range between $30,000 to $70,000 but one entry shows $500,000, this is a univariate outlier.
Multivariate Outliers
Definition: Outliers detected by analyzing relationships between multiple features (variables) simultaneously.
Example:
In a dataset with features "height" and "weight," a person with height = 200 cm and weight = 40 kg is a multivariate outlier since this combination is unusual despite individual values being normal.
Reply
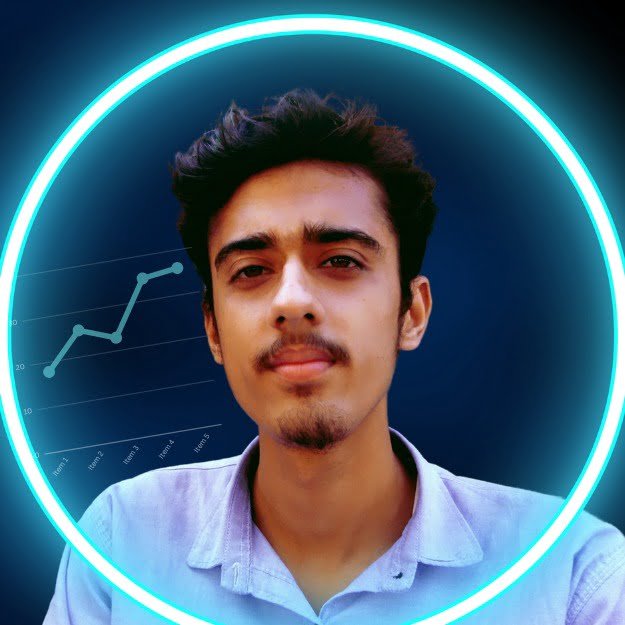
Inside the book of statistics, explanations for all the types of outliers are the same except univariate outliers.
Reply
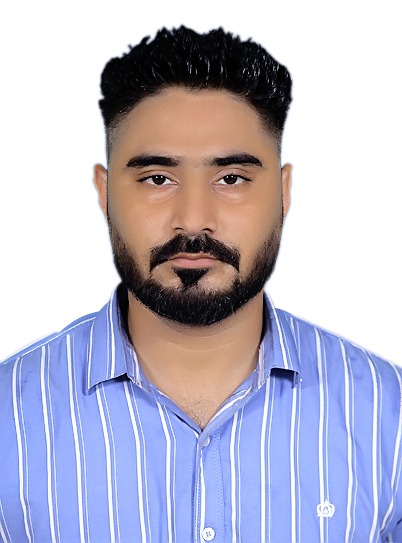
done once again
Reply
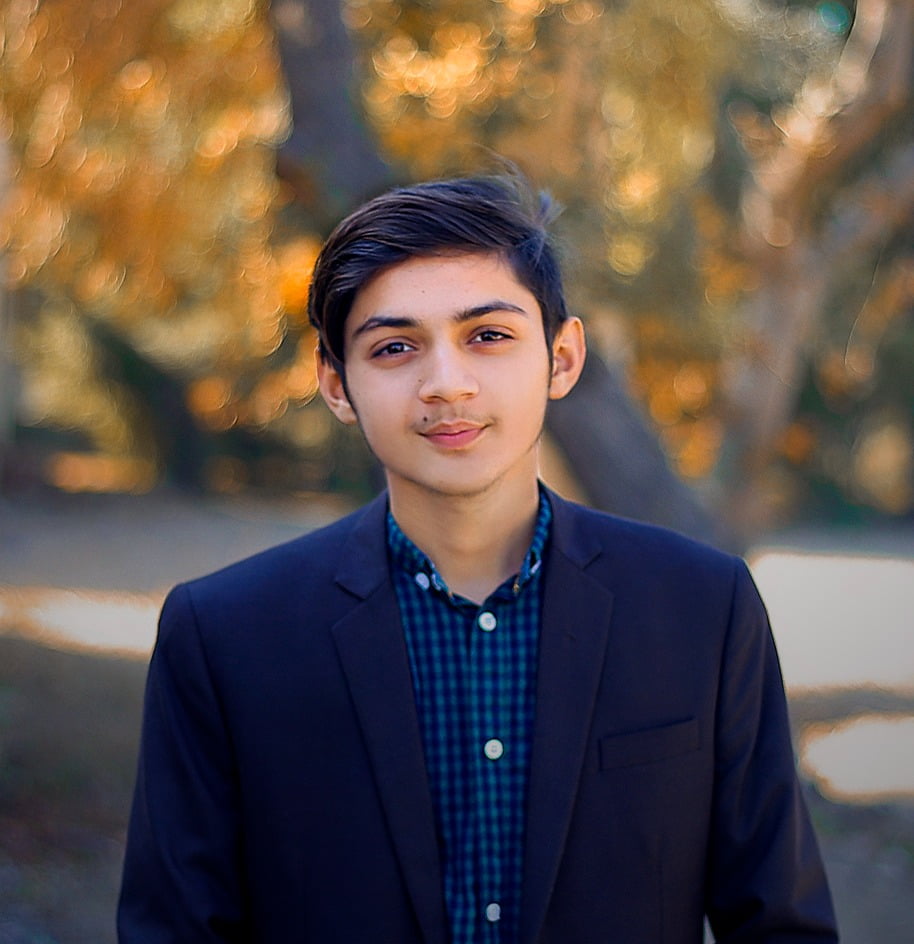
I learned about Outliers:
--> Outliers can:
1. divert the center, 2. cause Skewness, 3. Wrong insights, 4. Inefficient ML Model, 5. Wrong predictions.
--> Other names for Outliers:
Outliers, Deviants, Abnormalities, Anomalous points, Abberrvants observations.
-->Types of Outliers: Uni-variate, Bi-variate, Multi-variate, Global, Point, Local, Contextual.
--> How to handle Outliers:
1. Remove them 2. Transform them(log transform) 3. Impute with mean, median, mode 4. Seperate them and treat them separately 5. Use Robust Models
Reply
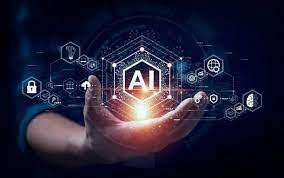
done
Reply
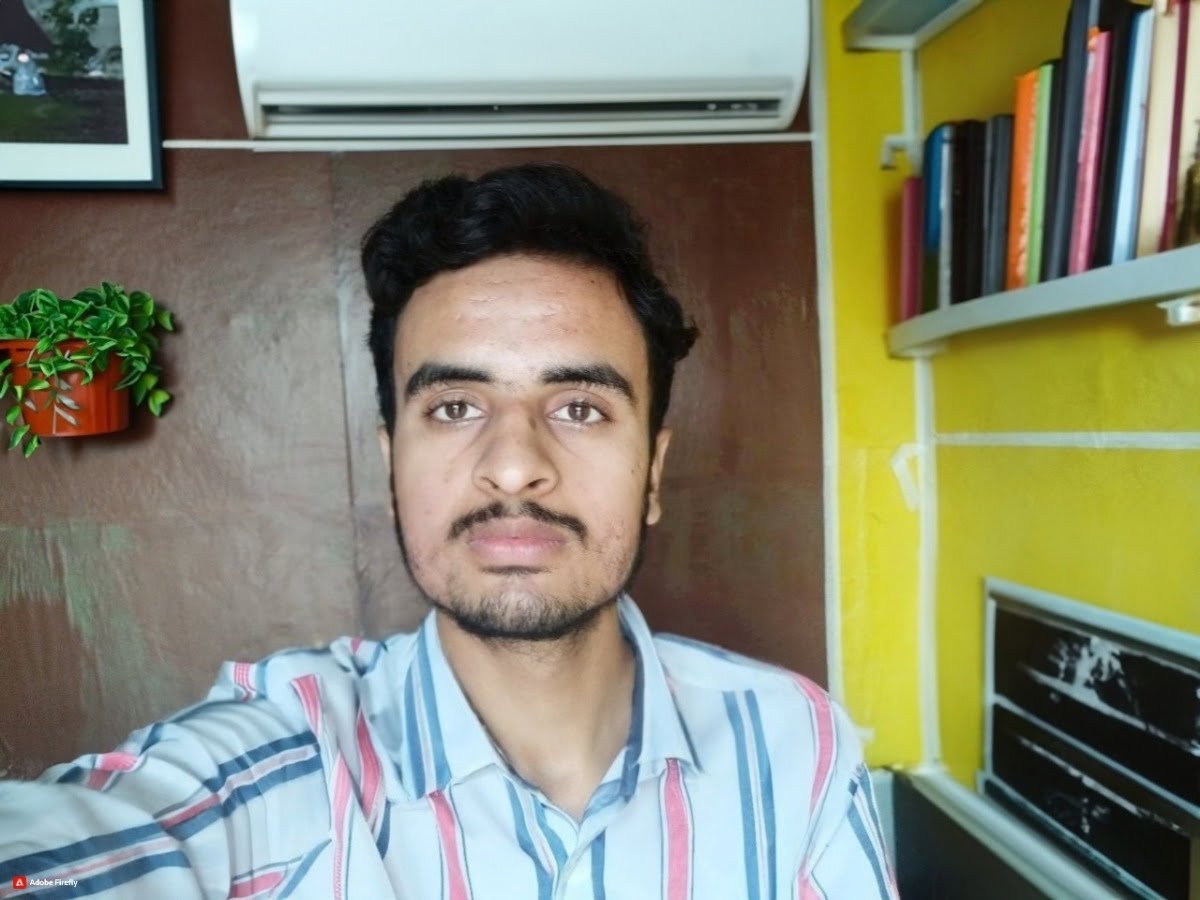
Done
Reply
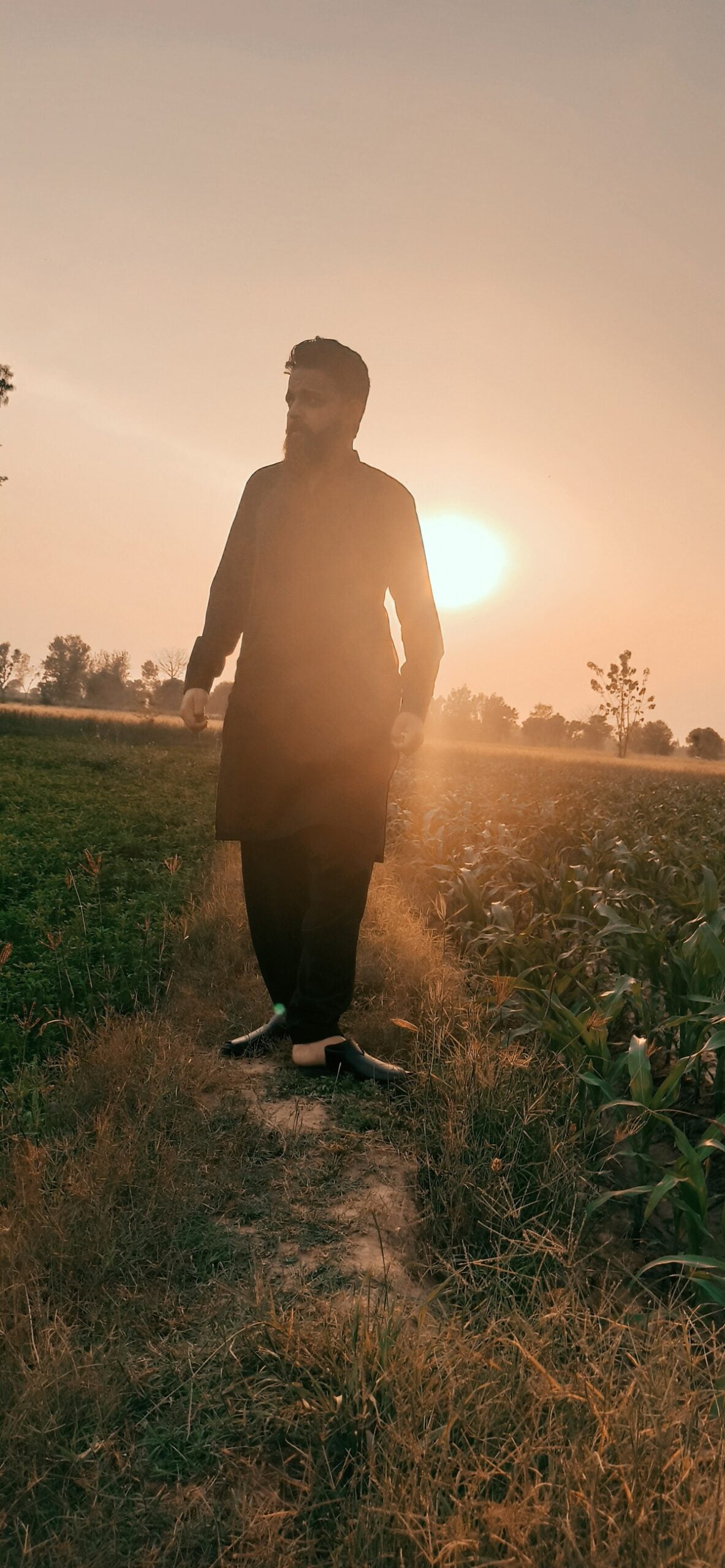
Done
Reply
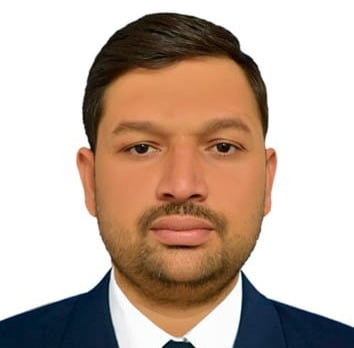
jazakumullah Kharn ameen
Reply
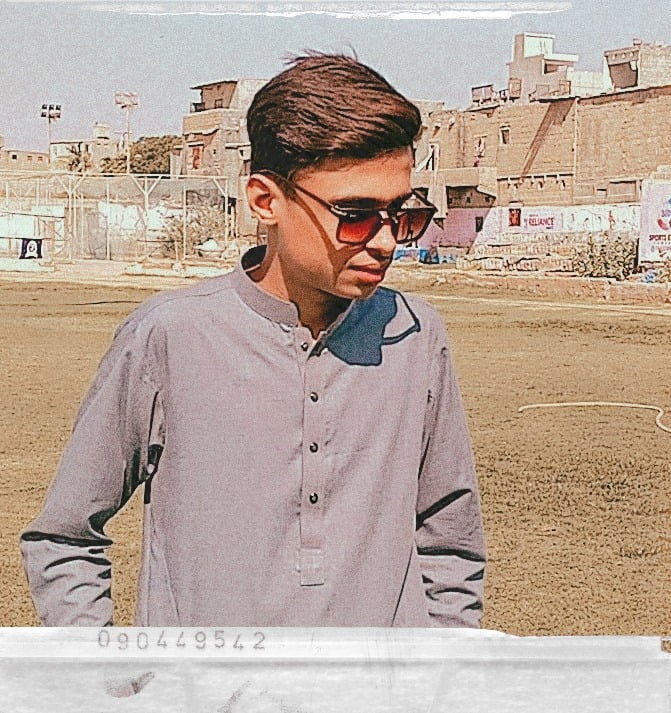
In this lecture, I have learned to extract outliers.
Reply