Join the conversation
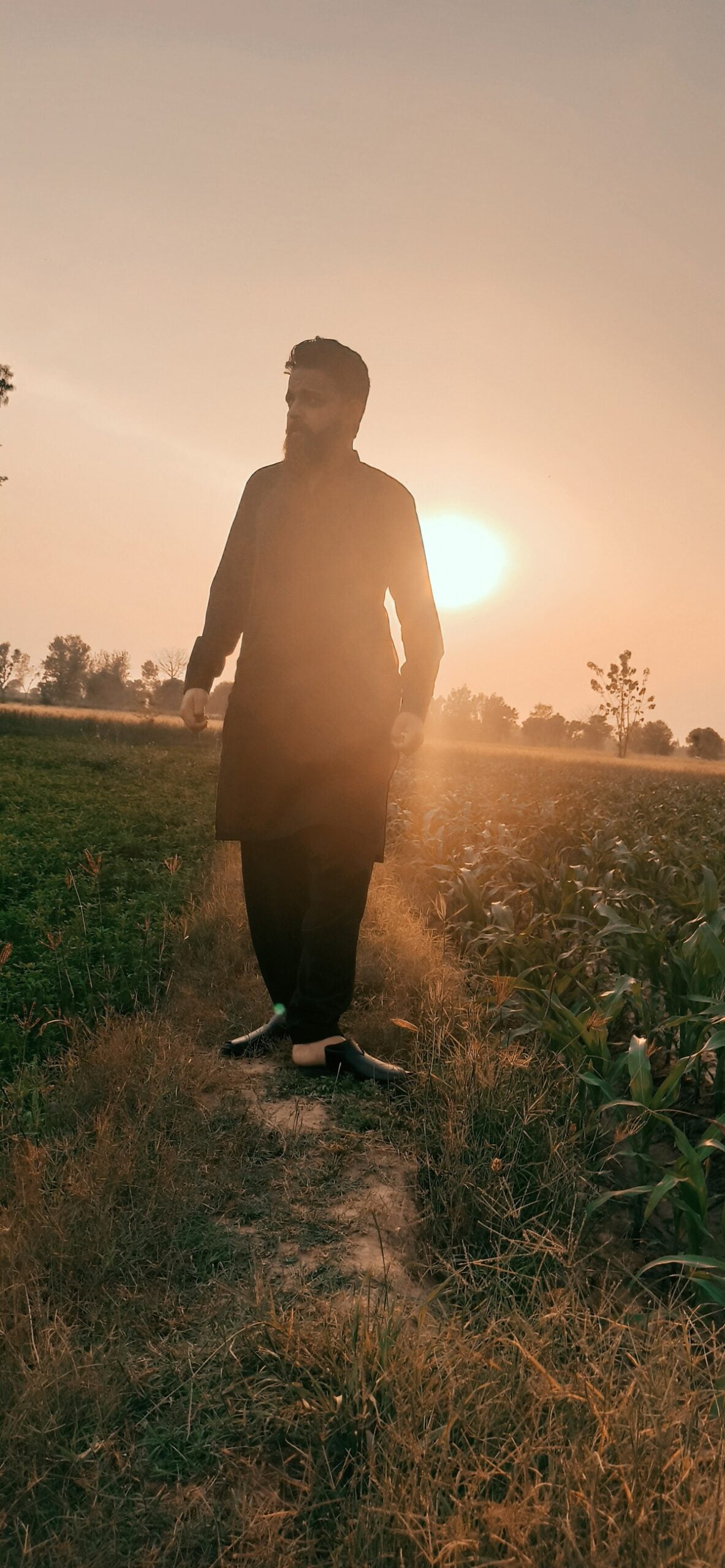
The main metrics used to evaluate logistic regression models are:1. Accuracy:The fraction of correct predictions out of the total number of predictions. It is calculated as (correct predictions) / (total predictions).
2. Precision: The fraction of true positive predictions out of all positive predictions. It measures how precise the model is at predicting the positive class.
3. Recall (Sensitivity): The fraction of true positive predictions out of all actual positive instances. It measures how well the model identifies positive instances.
4. F1-Score: The harmonic mean of precision and recall. It provides a balanced metric that considers both precision and recall.
ROC (Receiver Operating Characteristic) Curve: A plot of the true positive rate (recall) against the false positive rate (1 - specificity) at different classification thresholds. The AUC (Area Under the Curve) is a useful metric derived from the ROC curve.
5. Log Loss (Cross-Entropy Loss): The negative log-likelihood of the true labels given the predicted probabilities. It penalizes confident incorrect predictions more heavily.
Reply
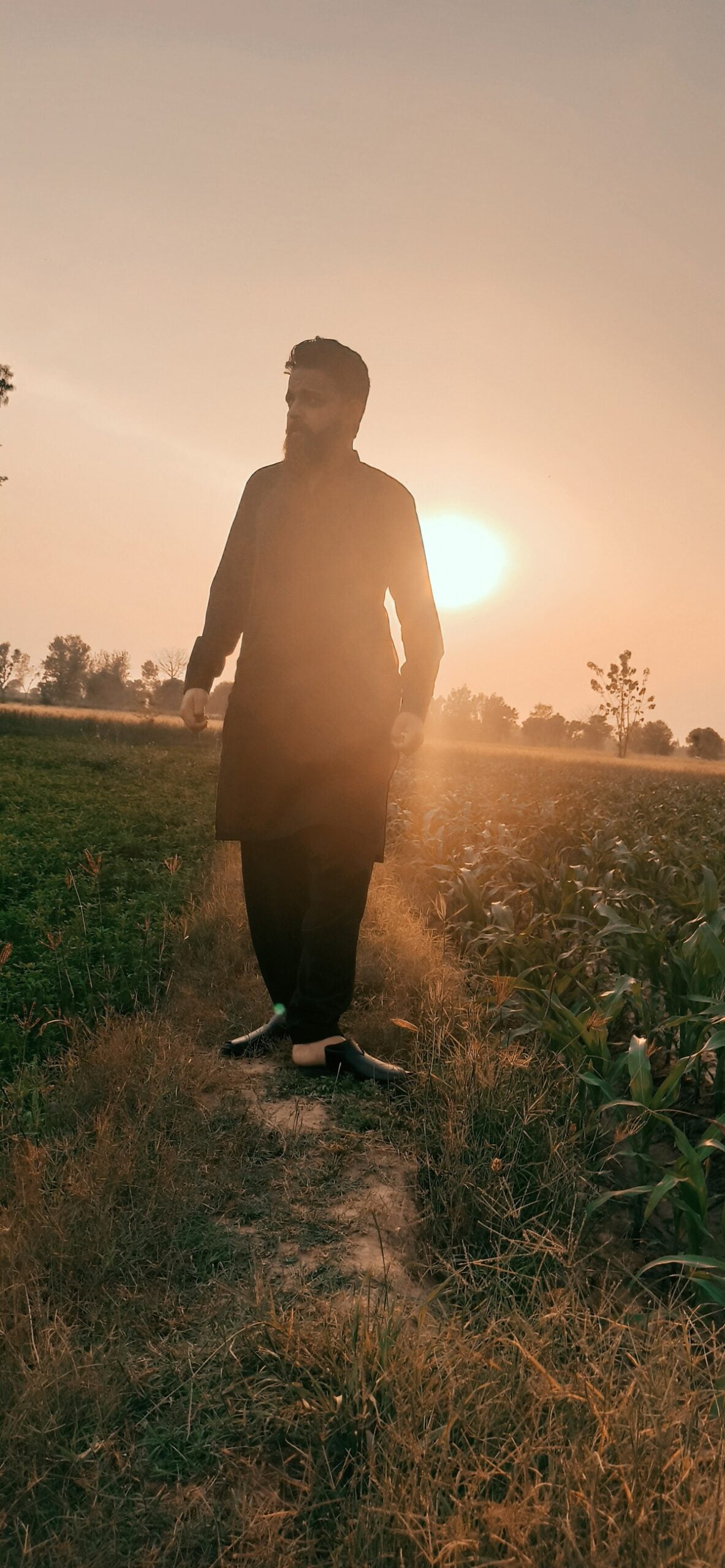
The main metrics used to evaluate logistic regression models are:
Accuracy: The fraction of correct predictions out of the total number of predictions. It is calculated as (correct predictions) / (total predictions).
Precision: The fraction of true positive predictions out of all positive predictions. It measures how precise the model is at predicting the positive class.
Recall (Sensitivity): The fraction of true positive predictions out of all actual positive instances. It measures how well the model identifies positive instances.
F1-Score: The harmonic mean of precision and recall. It provides a balanced metric that considers both precision and recall.
ROC (Receiver Operating Characteristic) Curve: A plot of the true positive rate (recall) against the false positive rate (1 - specificity) at different classification thresholds. The AUC (Area Under the Curve) is a useful metric derived from the ROC curve.
Log Loss (Cross-Entropy Loss): The negative log-likelihood of the true labels given the predicted probabilities. It penalizes confident incorrect predictions more heavily.
Reply
✍️done
Reply
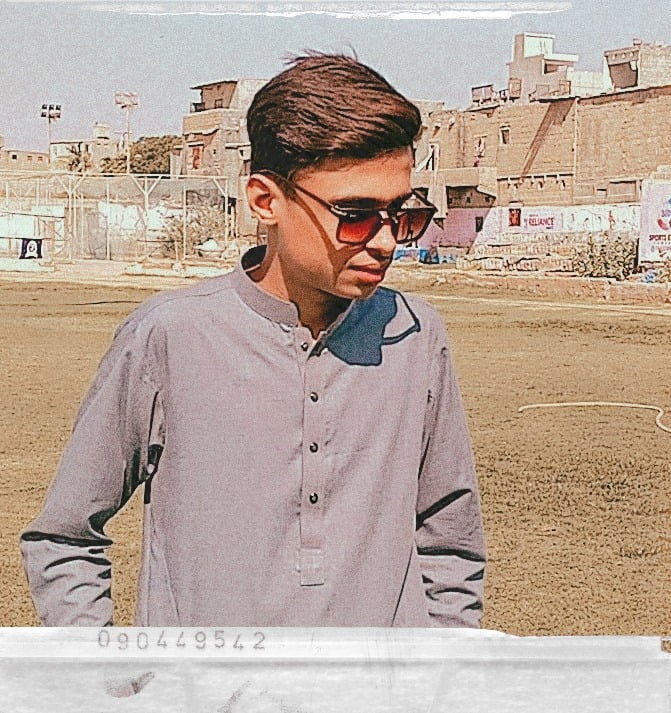
I learned in this lecture regression and classification matrics.
Reply
I learned in this lecture what methods would apply in finding the numerical data and/or categorical data.
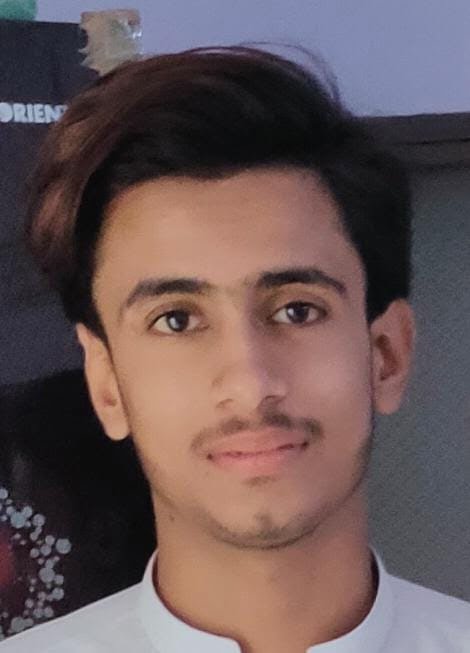
I learned Regression and Classification.
Reply
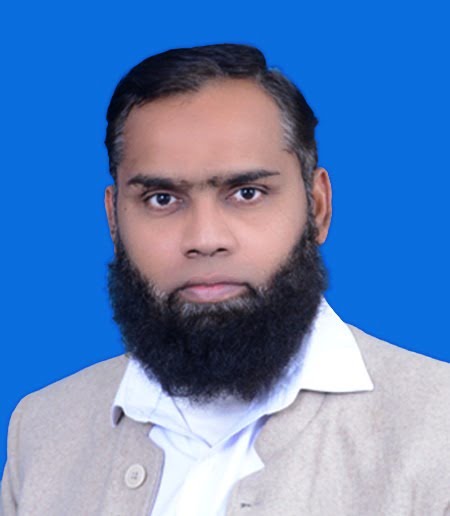
Regression and Classification Matrices overview discussed in this lecture. (1) Mean Squared Error - MSE (2) R2-Coefficient of Determination (3) Root Mean Squared Error (4) Mean Absolute Error (5) Accuracy Score (6) Recall Score (7) Precision Score (8) F1 Score (9) Confusion Matrix (10) Classification Report.
Reply
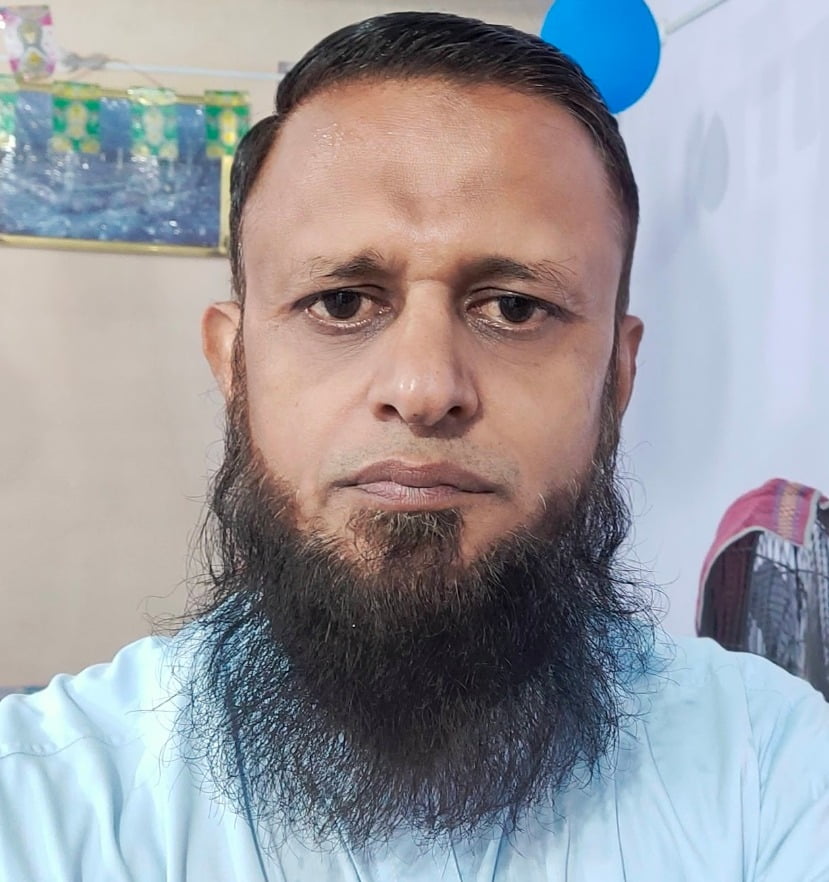
AOA, I learned in this lecture about the difference between regression and classification and what their basic metrics are.1. Regression ( numerical metrics, which are MSE, R^2, RMSE, and MAE )
2: Classification (categorical metrics, which are accuracy score, recall score, precision score, f1 score, and confusion matrix).
ALLAH PAK aap ko sahat o aafiat wali lambi umar ata kray aor ap ko dono jahan ki bhalian naseeb farmaey, Ameen.
Reply