Join the conversation
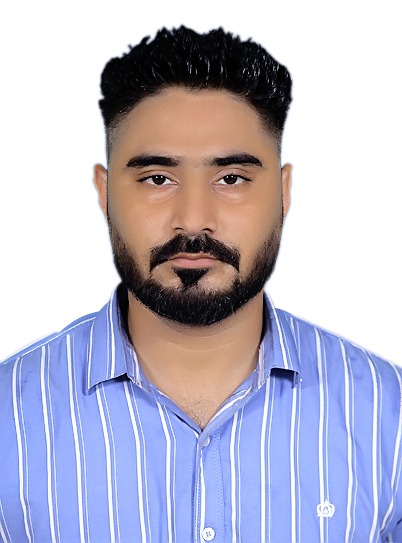
done
Reply
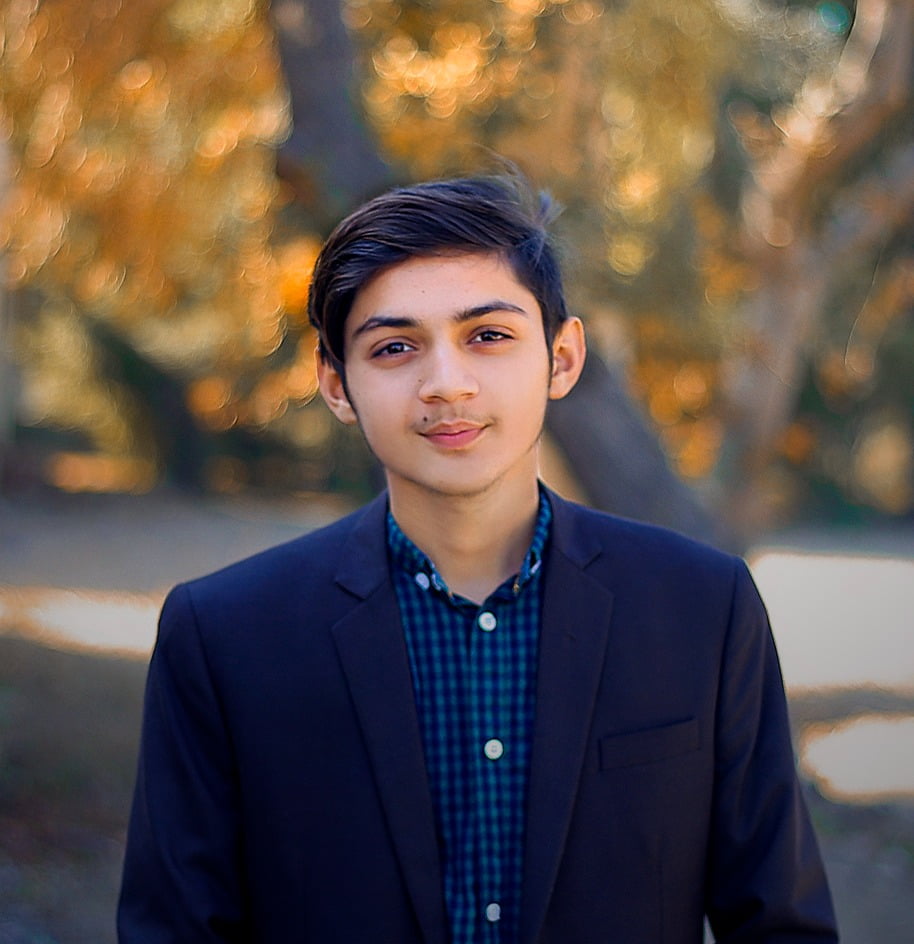
I learned about how to select the best models and how to do the hyperparameter Tuning on datasets.
Reply
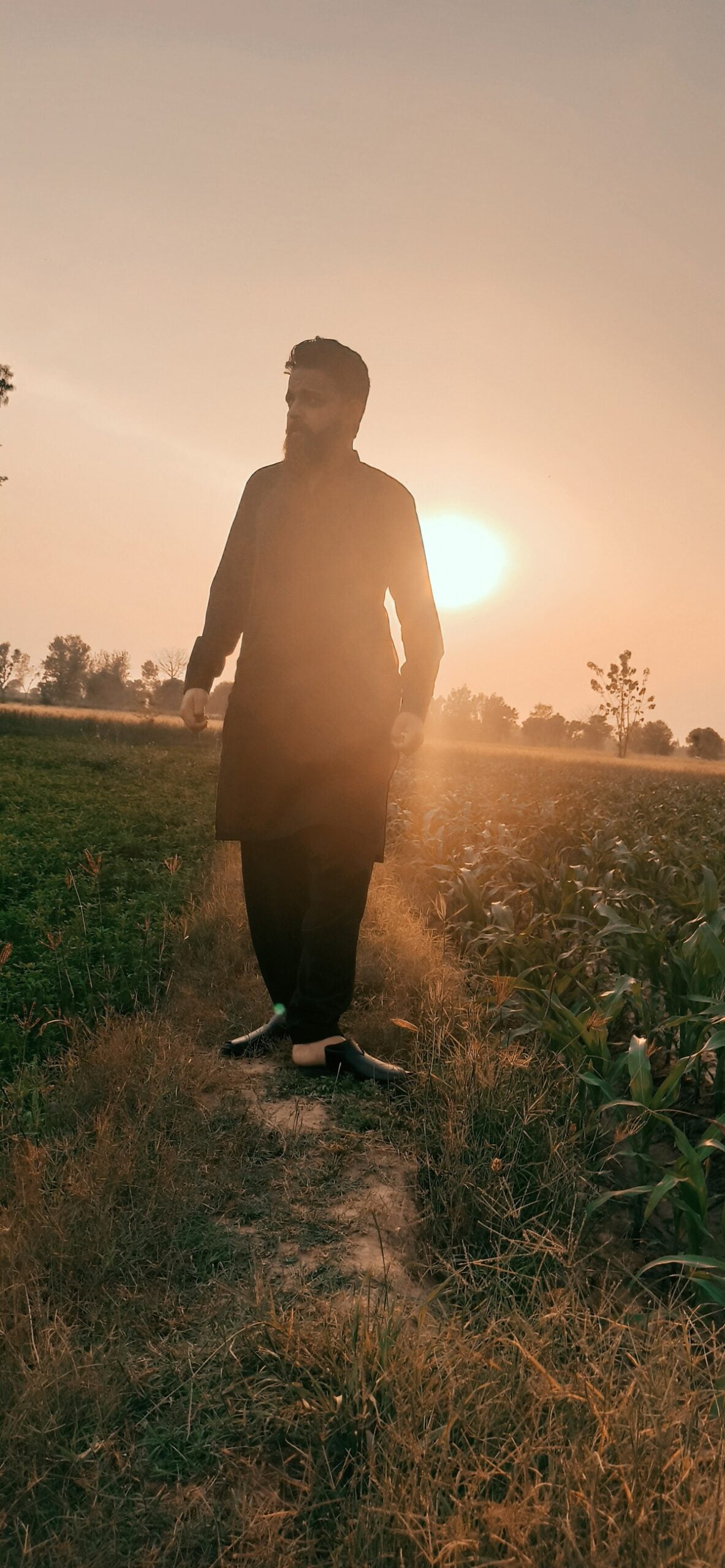
Done
Reply
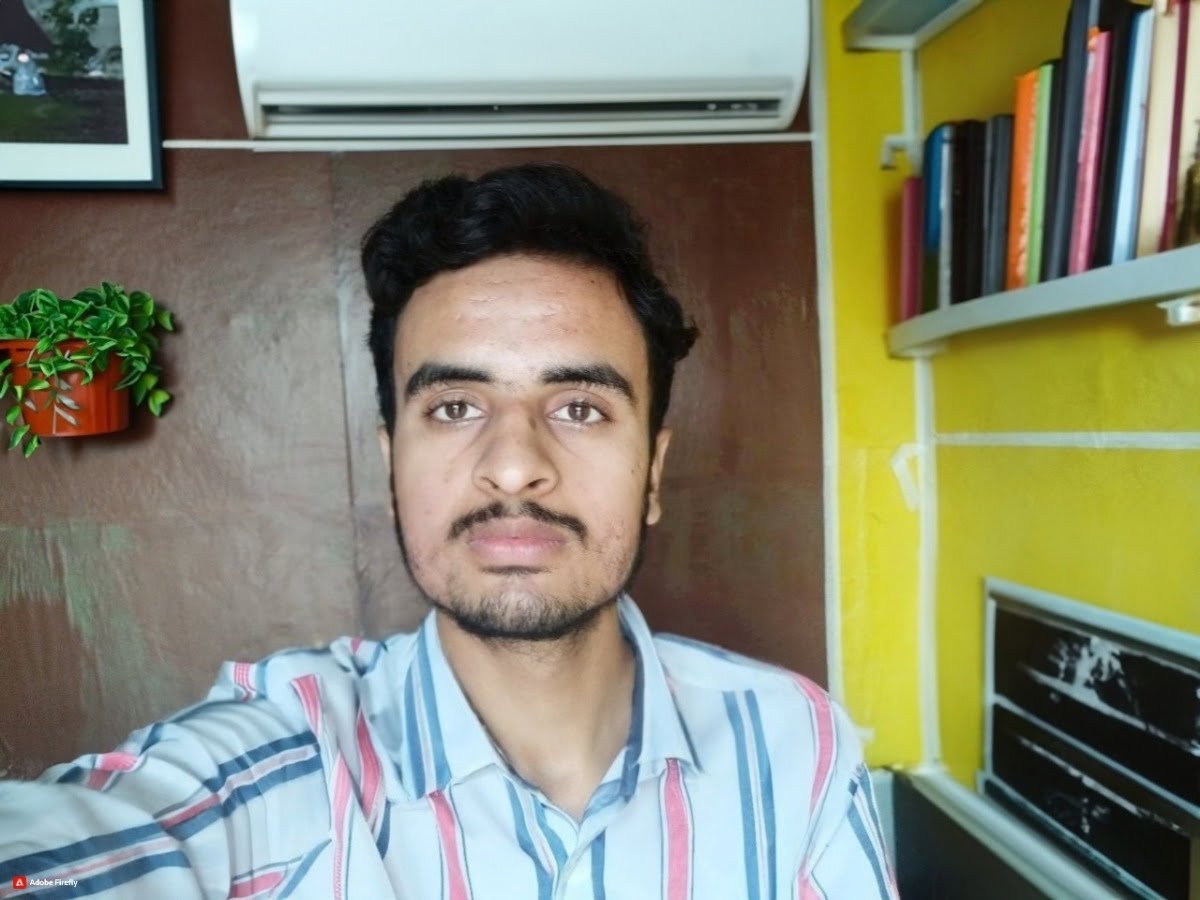
Done
Reply
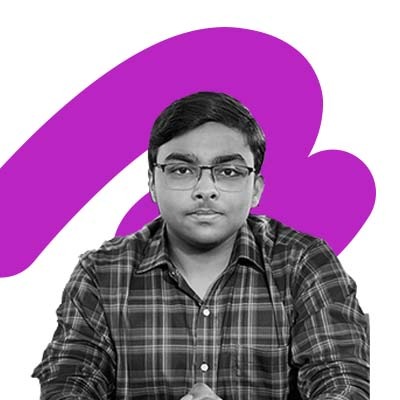
from sklearn.pipeline import Pipeline
from sklearn.model_selection import GridSearchCV
from sklearn.preprocessing import StandardScaler
from sklearn.linear_model import LinearRegression
from sklearn.tree import DecisionTreeRegressor
from sklearn.svm import SVR
from sklearn.ensemble import RandomForestRegressor# Define a list of models with their respective parameter grids
models = [
('LinearRegressor', Pipeline([
('preprocessor', StandardScaler()),
('model', LinearRegression())
]), {}),
('DecisionTreeRegressor', Pipeline([
('preprocessor', StandardScaler()),
('model', DecisionTreeRegressor())
]), {'model__max_depth': [None, 50, 100], 'model__criterion': ['mse', 'mae']}),
('SVR', Pipeline([
('preprocessor', StandardScaler()),
('model', SVR())
]), {'model__kernel': ['rbf', 'sigmoid'], 'model__C': [0.1, 1, 0.01]}),
('RandomForestRegressor', Pipeline([
('preprocessor', StandardScaler()),
('model', RandomForestRegressor())
]), {'model__max_depth': [None, 5, 10]})
]best_model = None
best_score = 0# Loop through each model and its parameter grid
for model_name, model, param_grid in models:
# Perform grid search if a parameter grid is provided
if param_grid:
grid_search = GridSearchCV(model, param_grid, cv=5)
grid_search.fit(X_train, y_train)best_params = grid_search.best_params_
best_model_score = grid_search.best_score_# print(f"Best parameters for {model_name}: {best_params}")
# print(f"Best score for {model_name}: {best_model_score}")if best_model_score > best_score:
best_model = grid_search.best_estimator_
best_score = best_model_score
else:
# Fit the model directly without grid search
model.fit(X_train, y_train)
score = model.score(X_train, y_train)print(f"Score for {model_name}: {score}")if score > best_score:
best_model = model
best_score = scoreprint(f"The best model is {best_model}")
Reply
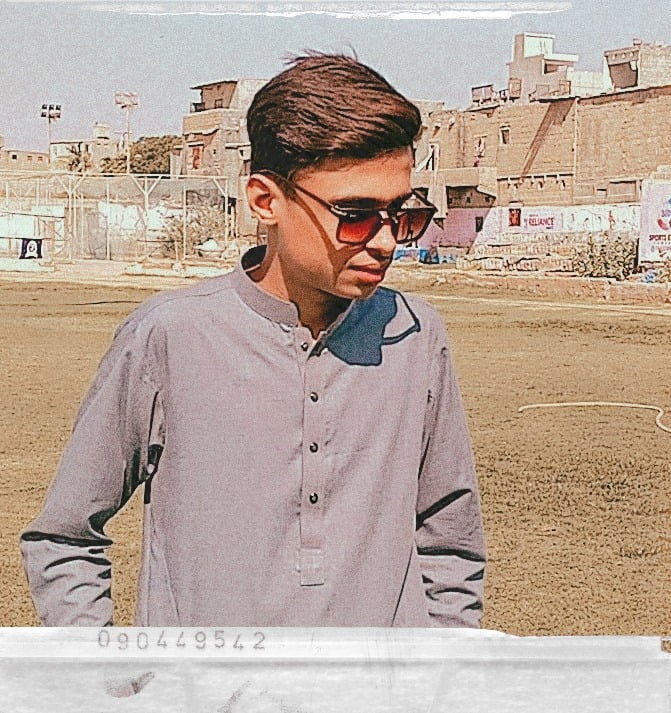
I have done this lecture with 100% practice.
Reply
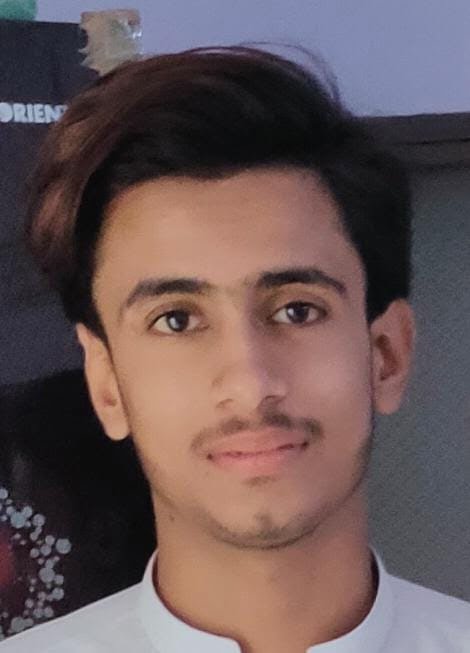
I have done this video with 100% practice.
Reply
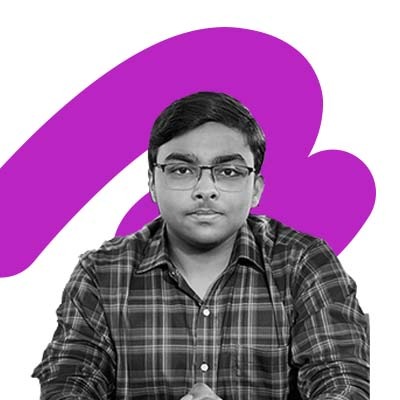
from sklearn.pipeline import Pipeline
from sklearn.model_selection import GridSearchCV
from sklearn.preprocessing import StandardScaler
from sklearn.linear_model import LinearRegression
from sklearn.tree import DecisionTreeRegressor
from sklearn.svm import SVR
from sklearn.ensemble import RandomForestRegressor# Define a list of models with their respective parameter grids
models = [
('LinearRegressor', Pipeline([
('preprocessor', StandardScaler()),
('model', LinearRegression())
]), {}),
('DecisionTreeRegressor', Pipeline([
('preprocessor', StandardScaler()),
('model', DecisionTreeRegressor())
]), {'model__max_depth': [None, 50, 100], 'model__criterion': ['mse', 'mae']}),
('SVR', Pipeline([
('preprocessor', StandardScaler()),
('model', SVR())
]), {'model__kernel': ['rbf', 'sigmoid'], 'model__C': [0.1, 1, 0.01]}),
('RandomForestRegressor', Pipeline([
('preprocessor', StandardScaler()),
('model', RandomForestRegressor())
]), {'model__max_depth': [None, 5, 10]})
]best_model = None
best_score = 0# Loop through each model and its parameter grid
for model_name, model, param_grid in models:
# Perform grid search if a parameter grid is provided
if param_grid:
grid_search = GridSearchCV(model, param_grid, cv=5)
grid_search.fit(X_train, y_train)best_params = grid_search.best_params_
best_model_score = grid_search.best_score_# print(f"Best parameters for {model_name}: {best_params}")
# print(f"Best score for {model_name}: {best_model_score}")if best_model_score > best_score:
best_model = grid_search.best_estimator_
best_score = best_model_score
else:
# Fit the model directly without grid search
model.fit(X_train, y_train)
score = model.score(X_train, y_train)print(f"Score for {model_name}: {score}")if score > best_score:
best_model = model
best_score = scoreprint(f"The best model is {best_model}")