Join the conversation
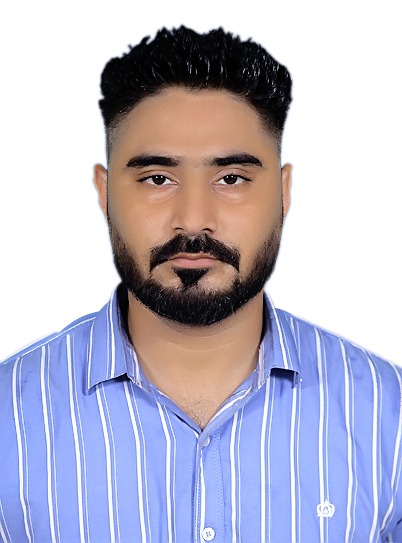
done classification metrics
Reply
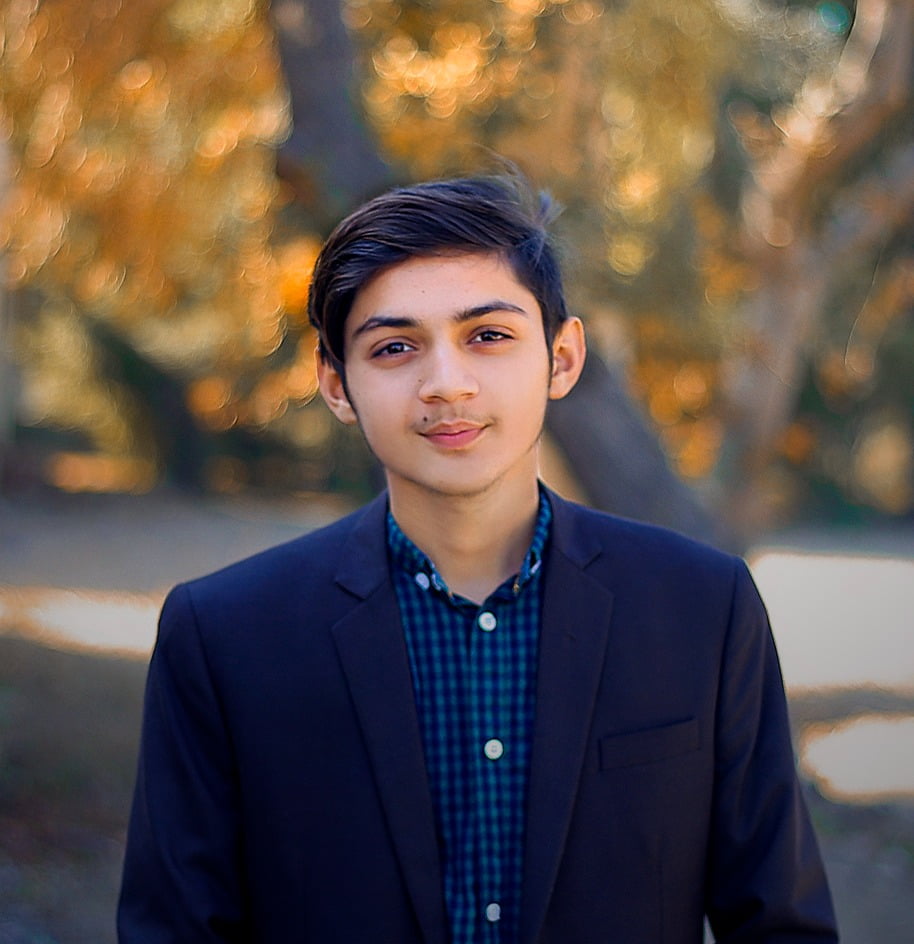
I learned about the Classification Evaluation Metrics:
Accuracy, Precision, Recall, F1-score, Area Under the Curve, Confusion Matrix
Reply
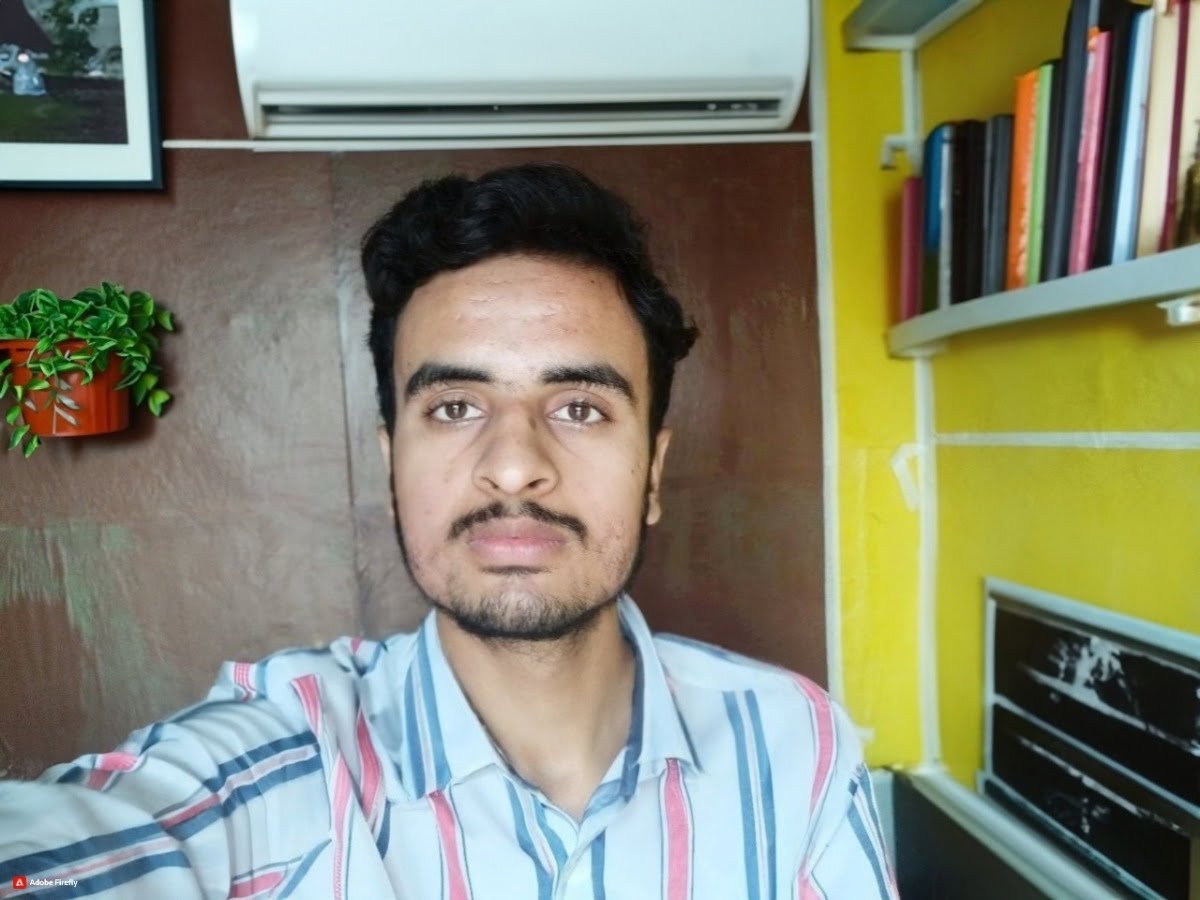
https://www.kaggle.com/muhammadrameez242 , ye meri kaggle ki I'd hai yha par Ap mukamal assignments simple roman urdu mein mill jai gi codes mein
Reply
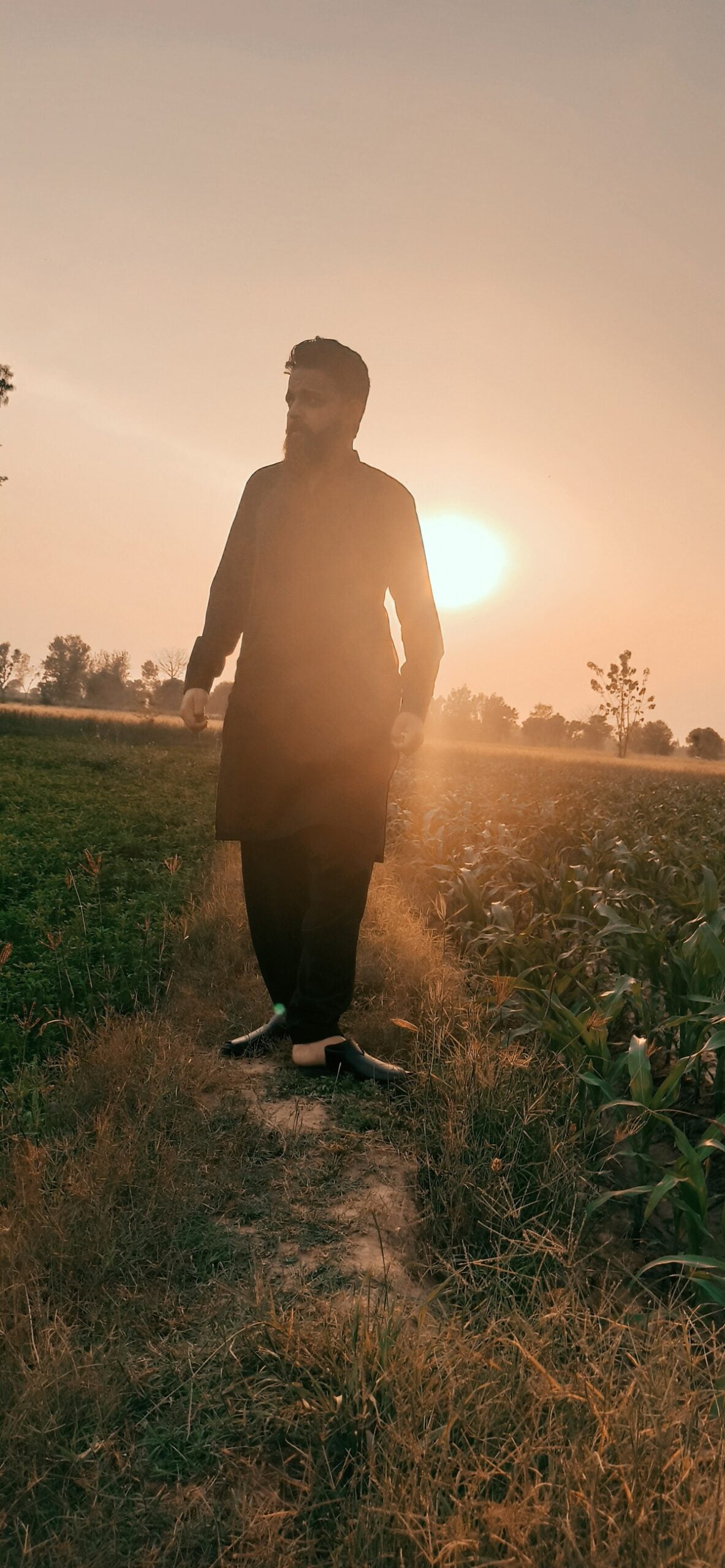
Done
Reply
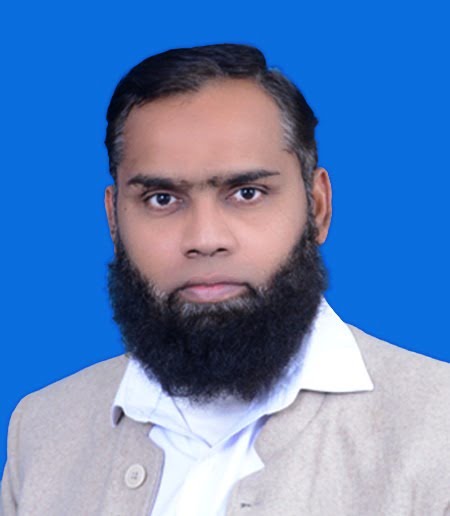
Very detailed understanding of evaluation metrics for classification techniques and formula usage in these metrics.
Reply
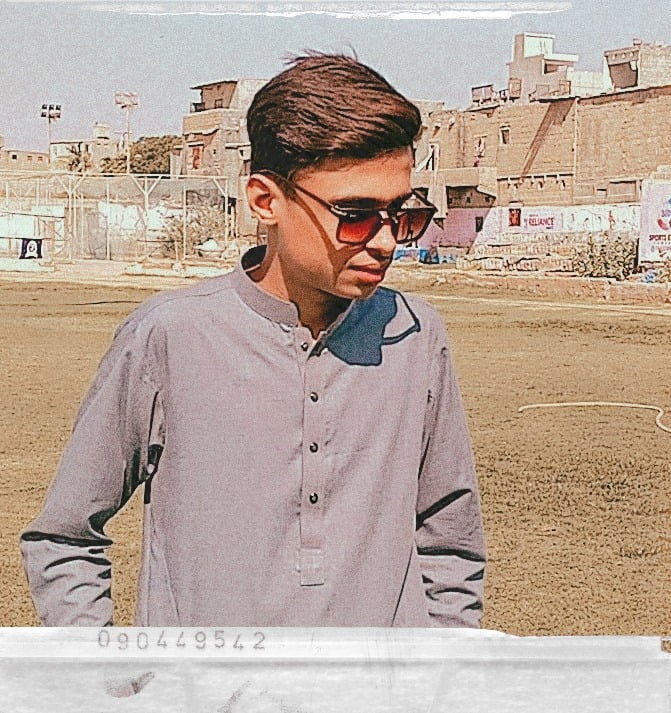
I learned in this lecture Accuracy, Precision, Recall, and F1 score. Confusion matrix.
Reply
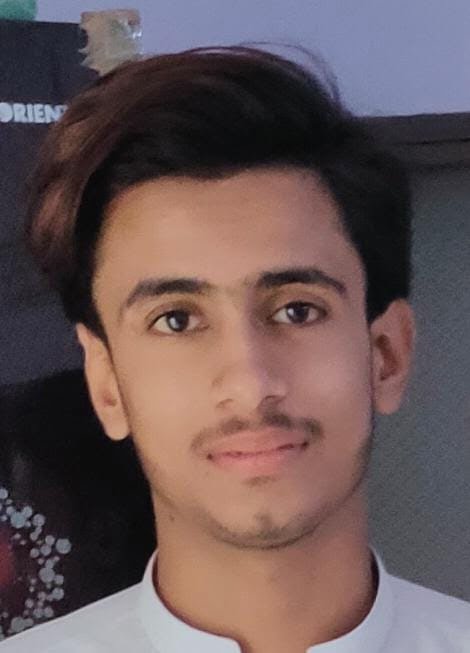
I learned in this video Accuracy, Precision, Recall, and F1 (Confusion metrics).
Reply
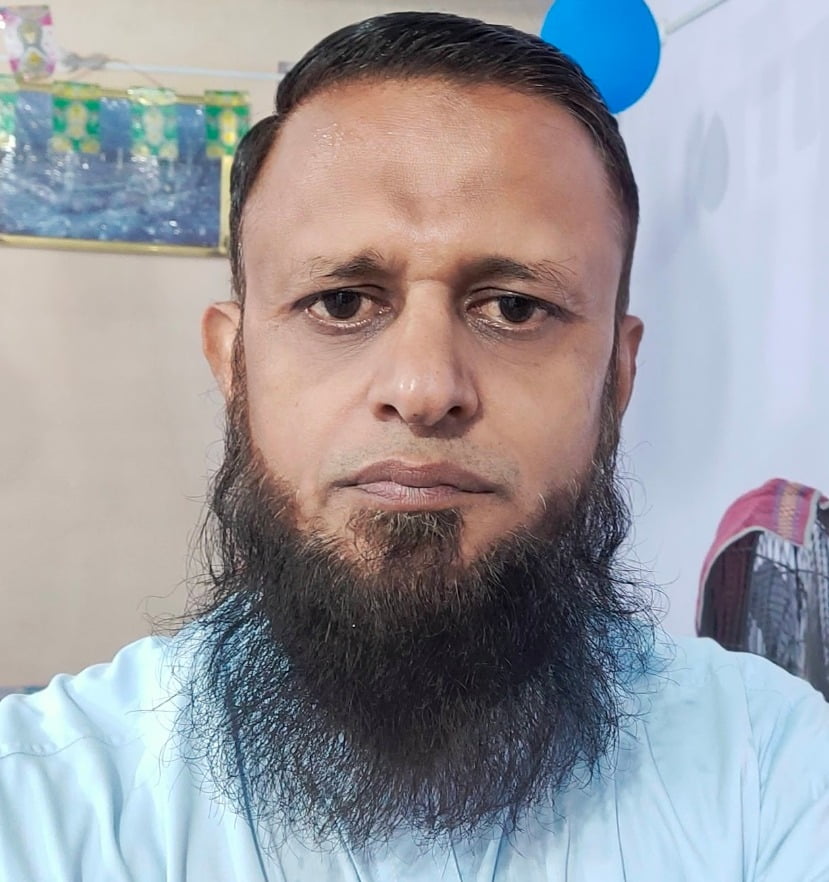
AOA, In this lecture, I learned how evaluation metrics help us measure model accuracy. We know that there are some types of machine learning algorithms and I also learned about Classification metrics which are1-Accuracy
Description: Proportion of correctly predicted observations to the total observations.
Pros: Simple and intuitive.
Cons: Can be misleading in imbalanced datasets.
Example: For 100 predictions with 90 correct predictions, accuracy is 90%2-Precision
Description: Proportion of correctly predicted positive observations to the total predicted positive observation.
Pros: It focuses on the relevancy of results.
Cons: Does not consider true negative results.
Example: For 30 true positive prediction out of 40 total positive predictions, precision is 75%3-Recall (Sensitivity)Description: Proportion of correctly predicted positive observations to all observation in actual class
Pros: It is useful in cases where False negatives are costly.
Cons: Can lead to ignoring the true negatives.
Example: For 30 true positive predictions out of 40 actual positive instances, recall is 75%
4-F1 ScoreDescription: Harmonic means of Precision and Recall.
Pros: Balances Precision and Recall.
Cons: May not be a good measure when there is an imbalance between precision and Recall
Example: With Precision =75% and Recall = 75% F1 Score is 2*(0.75*0.75)/(0.75+0.75) = 75%5-Area Under the ROC Curve (AUC-ROC)Description: Measures the ability of a classifier to distinguish between classes
Pros: Effective for binary classification problems
Cons: Less information for multi-class problems
Example: If the AUC is 0.90, there is a 90% chance that the model will be able to distinguish between positive and negative6-Confusion Matrix
Description: A table showing actual vs predicted values
Pros: Provides a detailed breakdown of correct and incorrect classification
Cons: More complex to interpret
Example: A matrix showing TP, FN, FP, and TNALLAH PAK aap ko sahat o aafiat wali lambi umar ata kray aor ap ko dono jahan ki bhalian naseeb farmaey or ap k walid-e-mohtram ko karwat karwat jannat ata farmay,Ameen.
Reply