Join the conversation

sirf accuracy ko evaluate karna ho to accuracy score use karein ga
Reply

1- Accuracy , 2-Recall , 3-Precision , 4-F1 Score , 5-Confusion Metrics
Reply
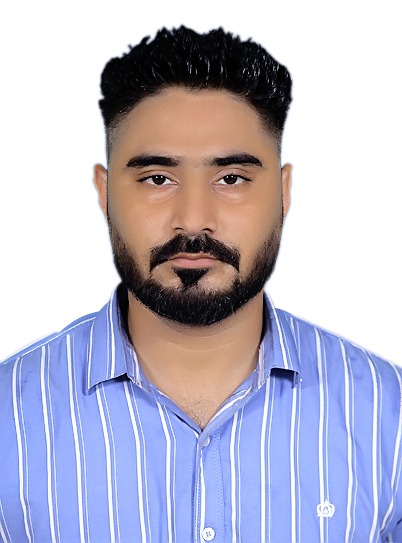
learn evluation metrics regression and classifition
Reply

Metrics of logostic regression(classification) are.....1- Accuracy,2-Recall,3-Precision,4-F1 score,5-confusion metrics
Reply
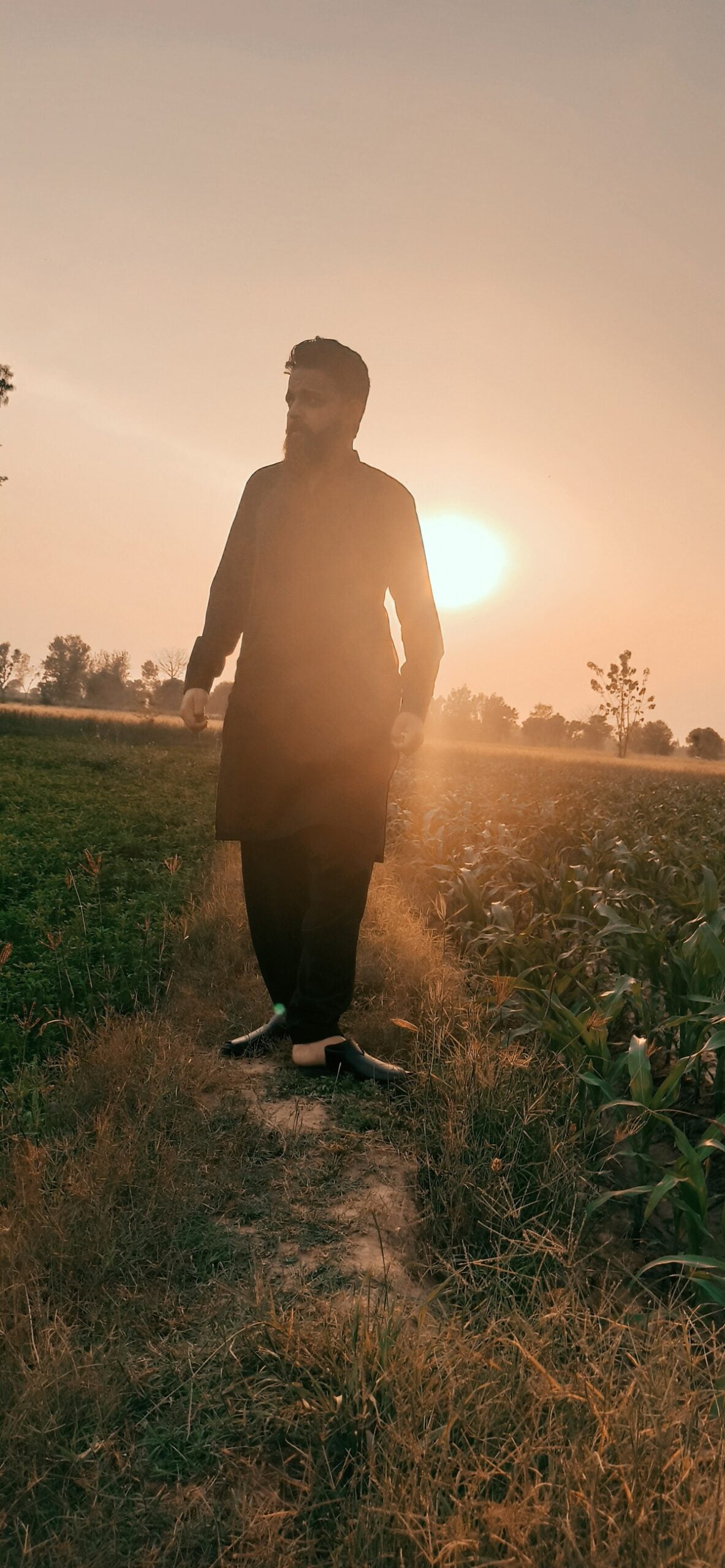
The main metrics used to evaluate logistic regression models are:1. Accuracy:The fraction of correct predictions out of the total number of predictions. It is calculated as (correct predictions) / (total predictions).
2. Precision: The fraction of true positive predictions out of all positive predictions. It measures how precise the model is at predicting the positive class.
3. Recall (Sensitivity): The fraction of true positive predictions out of all actual positive instances. It measures how well the model identifies positive instances.
4. F1-Score: The harmonic mean of precision and recall. It provides a balanced metric that considers both precision and recall.
ROC (Receiver Operating Characteristic) Curve: A plot of the true positive rate (recall) against the false positive rate (1 - specificity) at different classification thresholds. The AUC (Area Under the Curve) is a useful metric derived from the ROC curve.
5. Log Loss (Cross-Entropy Loss): The negative log-likelihood of the true labels given the predicted probabilities. It penalizes confident incorrect predictions more heavily.
Reply
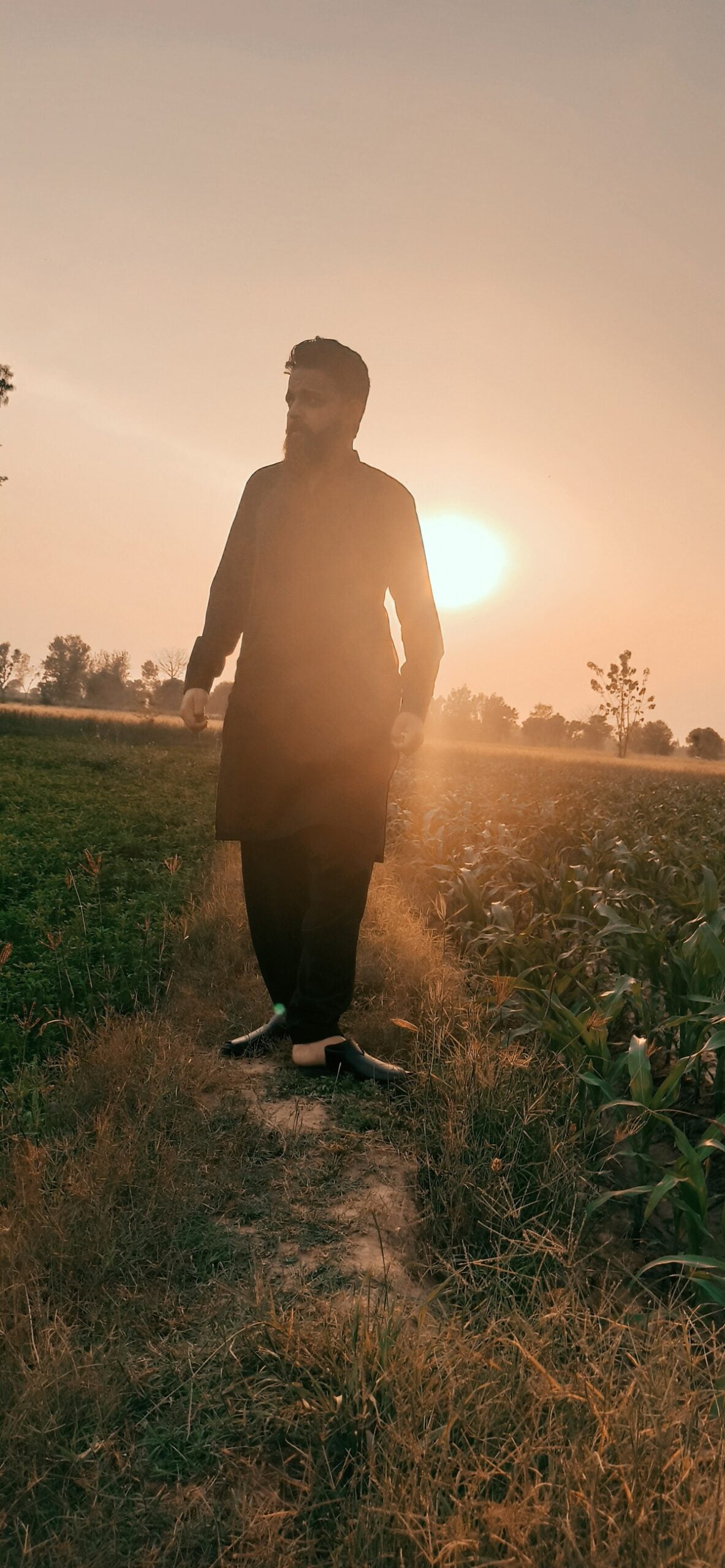
The main metrics used to evaluate logistic regression models are:
Accuracy: The fraction of correct predictions out of the total number of predictions. It is calculated as (correct predictions) / (total predictions).
Precision: The fraction of true positive predictions out of all positive predictions. It measures how precise the model is at predicting the positive class.
Recall (Sensitivity): The fraction of true positive predictions out of all actual positive instances. It measures how well the model identifies positive instances.
F1-Score: The harmonic mean of precision and recall. It provides a balanced metric that considers both precision and recall.
ROC (Receiver Operating Characteristic) Curve: A plot of the true positive rate (recall) against the false positive rate (1 - specificity) at different classification thresholds. The AUC (Area Under the Curve) is a useful metric derived from the ROC curve.
Log Loss (Cross-Entropy Loss): The negative log-likelihood of the true labels given the predicted probabilities. It penalizes confident incorrect predictions more heavily.
Reply

✍️done
Reply
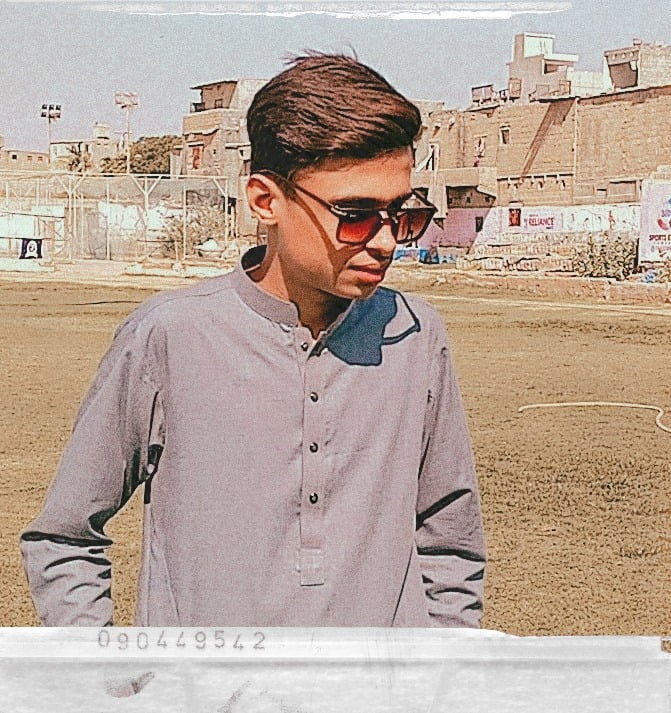
I learned in this lecture regression and classification matrics.
Reply

I learned in this lecture what methods would apply in finding the numerical data and/or categorical data.
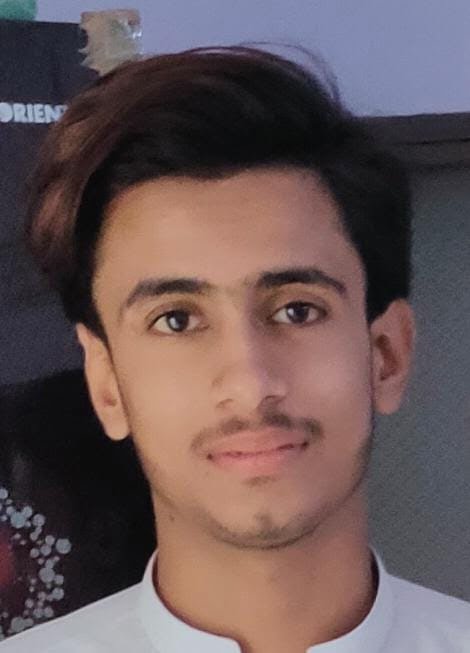
I learned Regression and Classification.
Reply
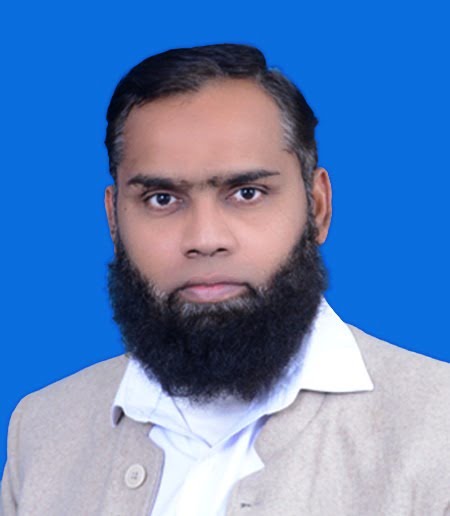
Regression and Classification Matrices overview discussed in this lecture. (1) Mean Squared Error - MSE (2) R2-Coefficient of Determination (3) Root Mean Squared Error (4) Mean Absolute Error (5) Accuracy Score (6) Recall Score (7) Precision Score (8) F1 Score (9) Confusion Matrix (10) Classification Report.
Reply