Join the conversation

1. Label Encoding
Description: Converts categorical values into numerical form by assigning each unique category an integer.
Use Case:
Best for ordinal categorical variables (e.g., "low," "medium," "high"), where the order matters.
Not suitable for nominal variables (e.g., "red," "blue," "green") as it may introduce an arbitrary order.
2. One-Hot Encoding
Description: Converts each category value into a new binary (0 or 1) column.
Use Case:
Best for nominal categorical variables where no ordinal relationship exists.
Helps prevent models from assuming a particular order or relationship among categories.
3. Binary Encoding
Description: Converts categories into binary code and creates as many columns as needed for the highest integer in the binary representation.
Use Case:
Useful when there are many categories, reducing the dimensionality compared to one-hot encoding.
Suitable when the categories are nominal.
4. Target Encoding (Mean Encoding)
Description: Replaces categories with the mean of the target variable for each category.
Use Case:
Effective for high-cardinality categorical variables, especially in regression tasks.
Should be used cautiously as it may introduce overfitting; proper cross-validation is needed.
5. Count Encoding
Description: Replaces categories with the count of occurrences in the dataset.
Use Case:
Useful when the frequency of the category has predictive power.
Can be used for both nominal and ordinal data.
6. Frequency Encoding
Description: Similar to count encoding but replaces categories with their relative frequency instead of the raw count.
Use Case:
Helps when the absolute count is not as important as the proportion of categories.
Can be used for nominal variables.
7. Ordinal Encoding
Description: Similar to label encoding but explicitly considers the order of categories.
Use Case:
Used for ordinal categories where the order matters (e.g., ratings).
Considerations for Choosing the Right Encoding:
Nature of Data: Determine whether the categorical variable is nominal or ordinal.
Model Requirements: Some models (e.g., tree-based models) can handle categorical variables naturally, while others (e.g., linear models) may require numerical input.
Cardinality: High cardinality (many unique values) may influence the choice of encoding due to increased dimensionality.
Risk of Overfitting: Techniques like target encoding can increase the risk of overfitting; using techniques like cross-validation is advisable.
By understanding these encoding techniques and their appropriate use cases, you can better prepare your categorical data for machine learning models.
Reply

Encoding Type Use Case
Label Encoding Ordinal data where order is meaningful.
One-Hot Encoding Nominal data with a small number of unique categories.
Ordinal Encoding Ordinal data where the order matters, but the difference between categories does not.
Target Encoding Strong relationship between category and target variable; works well with regularization.
Frequency Encoding High-cardinality data where frequency is important.
Binary Encoding High-cardinality categorical data.
Hash Encoding Very high-cardinality data in large-scale systems.
Dummy Encoding Avoiding multicollinearity in linear models.
Reply
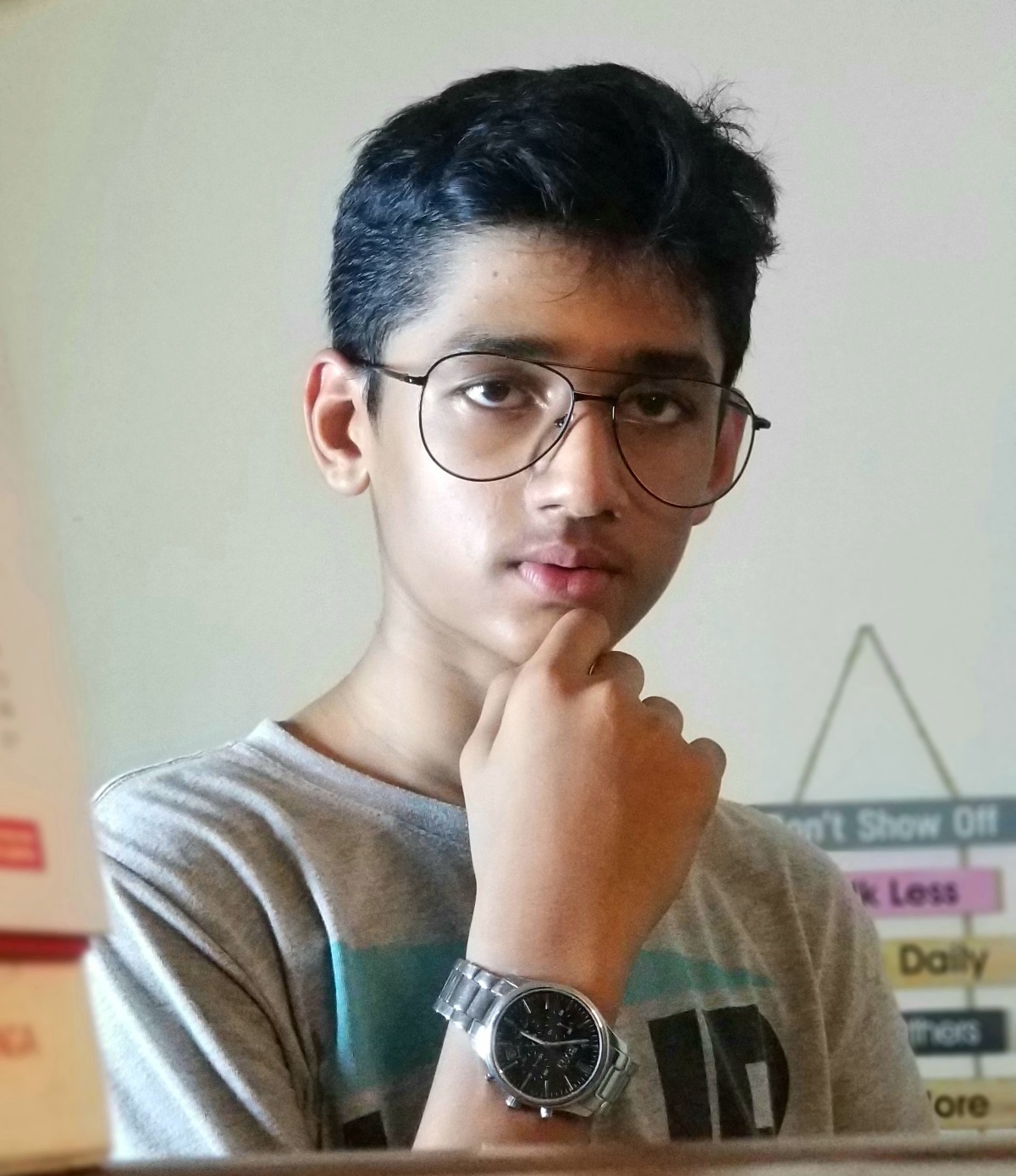
U CAN USE DF.SAMPLE TO GET EVERY VALUE OF THE COLUMN
Reply
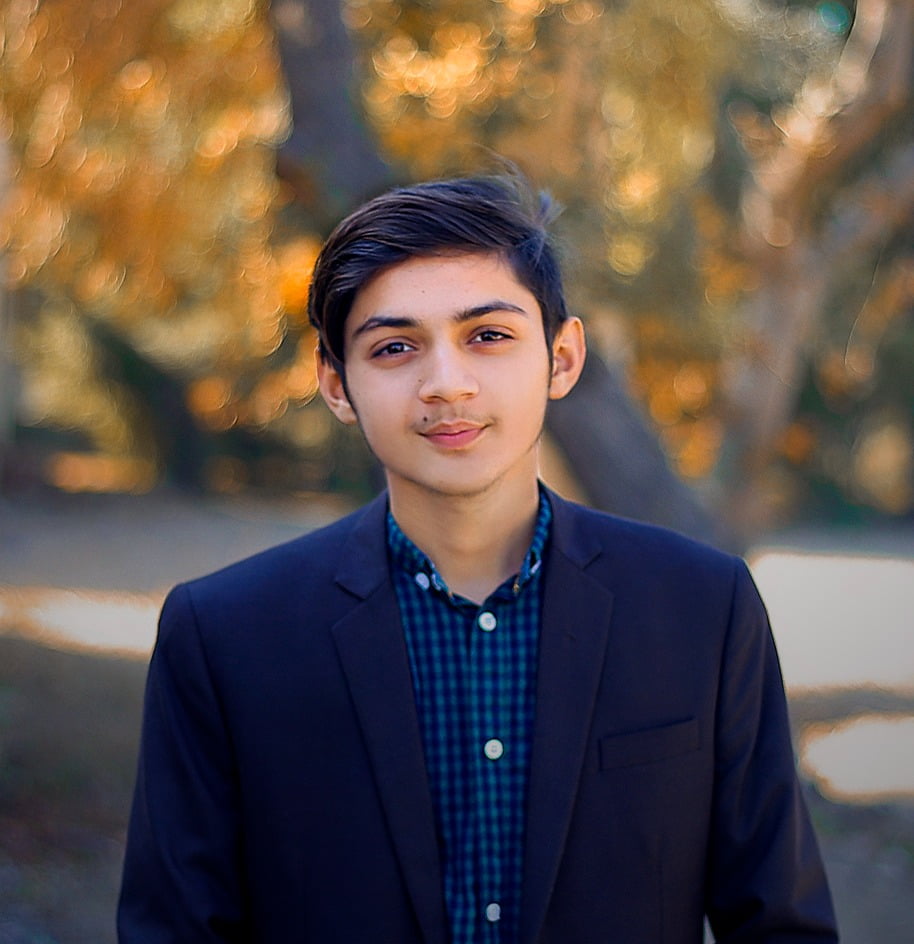
Assignment: (GPT response but very helpful)
### Q1: Different Types of Feature Encoding TechniquesFeature encoding is the process of converting categorical data into numerical data so that machine learning algorithms can process it. Here are different types of feature encoding techniques:1. **Label Encoding**
2. **One-Hot Encoding**
3. **Binary Encoding**
4. **Ordinal Encoding**
5. **Frequency Encoding**
6. **Target Encoding**
7. **Hash Encoding**
8. **Leave-One-Out Encoding****Most Important and Famous Ones:**1. **Label Encoding:**
- Converts each unique category to a numerical value.
- Simple and easy to implement.
- Used for ordinal data where there is an inherent order.2. **One-Hot Encoding:**
- Converts categories into binary columns.
- No ordinal relationship assumed.
- Suitable for nominal data.3. **Binary Encoding:**
- Reduces dimensionality compared to one-hot encoding.
- Each category is converted into binary and then split into columns.4. **Ordinal Encoding:**
- Assigns numerical values based on order.
- Used for ordinal data with a clear ranking.5. **Frequency Encoding:**
- Encodes categories based on the frequency of their occurrence.
- Useful for dealing with high cardinality features.6. **Target Encoding:**
- Encodes categories based on the mean of the target variable.
- Can introduce leakage; needs careful handling.### Q2: Which Feature Encoding Techniques to Use and When1. **Label Encoding:**
- **Use When:** You have ordinal data with a meaningful order (e.g., ratings, ranks).
- **Example:** ['low', 'medium', 'high'] → [0, 1, 2]2. **One-Hot Encoding:**
- **Use When:** You have nominal data without an inherent order.
- **Example:** ['red', 'blue', 'green'] → [ [1, 0, 0], [0, 1, 0], [0, 0, 1] ]3. **Binary Encoding:**
- **Use When:** You have high cardinality categorical features and want to reduce dimensionality.
- **Example:** ['cat', 'dog', 'mouse'] → [ ['cat'] → 001, ['dog'] → 010, ['mouse'] → 011]4. **Ordinal Encoding:**
- **Use When:** There is a clear, meaningful order in the categories.
- **Example:** ['first', 'second', 'third'] → [1, 2, 3]5. **Frequency Encoding:**
- **Use When:** Dealing with high cardinality features and you want to use the frequency information.
- **Example:** ['apple', 'banana', 'apple', 'apple', 'banana'] → [3, 2, 3, 3, 2]6. **Target Encoding:**
- **Use When:** You want to capture the relationship between categorical feature and target variable (especially in regression tasks).
- **Example:** Encoding 'city' based on the average house prices in that city.7. **Hash Encoding:**
- **Use When:** You need to handle very high cardinality and want a fixed-size encoding.
- **Example:** Using a hash function to map categories to a fixed number of columns.8. **Leave-One-Out Encoding:**
- **Use When:** You want to mitigate target leakage in target encoding by excluding the current row when calculating the mean.
- **Example:** For each category, calculate the mean of the target variable excluding the current instance.Choosing the right encoding technique depends on the nature of your data and the specific requirements of your machine learning model.
Reply

1. Ordinal Encoding
Use Case: Categorical variables with inherent order or ranking.
Example: ["Low", "Medium", "High"] could be encoded as [1, 2, 3].
2. One-Hot Encoding
Use Case: Nominal categorical variables with no inherent order.
Example: ["Red", "Blue", "Green"] could be encoded as three separate binary columns: Red (1, 0, 0), Blue (0, 1, 0), Green (0, 0, 1).
3. Binary Encoding
Use Case: High-cardinality nominal categorical variables.
Example: "Category 15" could be encoded to binary and then split into separate columns.
4. Label Encoding
Use Case: Categorical variables with a meaningful ordinal relationship.
Example: ["First", "Second", "Third"] could be encoded as [1, 2, 3].
5. Count Encoding
Use Case: When the frequency of occurrences of a category is relevant.
Example: A category that appears 10 times in the dataset would be encoded as 10.
6. Target Encoding / Mean Encoding
Use Case: When the relationship between the categorical variable and the target variable is important.
Example: Encoding categories based on the mean of the target variable for each category.
7. Frequency Encoding
Use Case: When the frequency of categories is relevant.
Example: A category appearing 5% of the time would be encoded as 0.05.
8. Feature Hashing
Use Case: Dealing with high-cardinality categorical features to reduce dimensionality.
Example: Hashing each category into a fixed number of columns.
9. Embedding Layers
Use Case: Embedding layers in neural networks for categorical variables.
Example: Mapping each category to a dense vector representation within the network.
10. Entity Embeddings of Categorical Variables
Use Case: Learning dense representations of categorical variables in deep learning scenarios.
Example: Similar to embedding layers, used to capture relationships between categories in a low-dimensional space.
Brief Descriptions:
A. Ordinal Encoding: Used for categorical variables with inherent order or ranking.
B. One-Hot Encoding: Used for nominal categorical variables without inherent order.
C. Binary Encoding: Used with high-cardinality nominal categorical variables.
D. Label Encoding: Used when the ordinal relationship between categories is known and meaningful.
E. Count Encoding: Used when the frequency of occurrences of a category is relevant information.
F. Target Encoding / Mean Encoding: Used when the relationship between the categorical variable and the target variable is important.
G. Frequency Encoding: Used when the frequency of categories is relevant.
H. Feature Hashing: Used when dealing with high-cardinality categorical features to reduce dimensionality.
J. Embedding Layers: Used for embedding layers in neural networks for categorical variables.
K. Entity Embeddings of Categorical Variables: Useful in deep learning scenarios for learning dense representations of categorical variables.
These encoding methods help transform categorical data into numerical formats suitable for machine learning models.
Reply
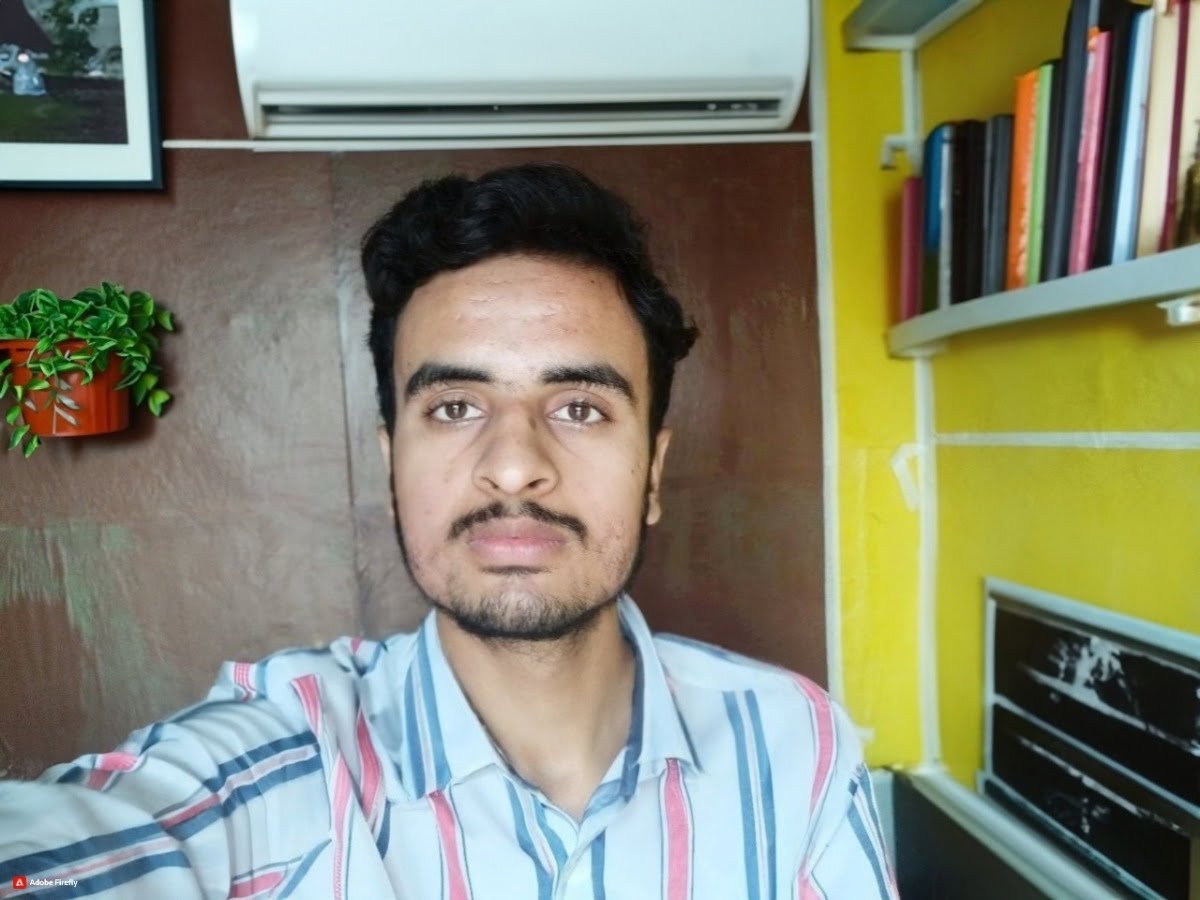
Done
Reply
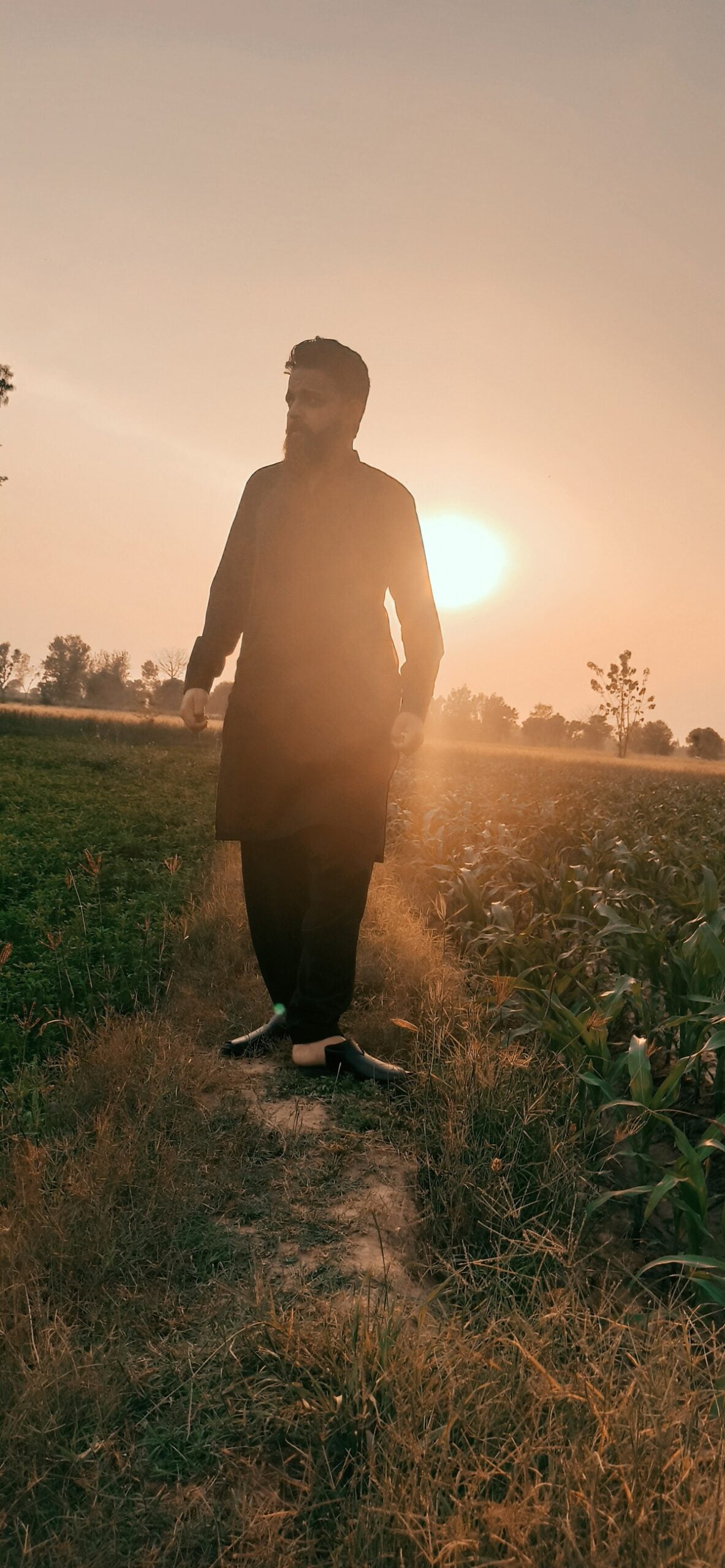
Done
Reply

1. Label Encoding: Assigns unique label to each category, used for ordinal data where the order matters.
2. On-Hot Encoding: Creates binary columns for each category, indicating the presence or absence. Best for nominal data and works well when the number of categories is not too high.
3. Ordinal Encoding: Assigns numerical values based on the order. Useful for ordinal data when we have a clear order among categories.
4. Binary Encoding: Converts categories into binary code. Efficient when dealing with high cardinality categorical features.
5. Frequency Encoding: Uses the frequency of each category as its representation, works when categories with higher frequencies might carry more significance.
6. Target Encoding: Involves replacing a categorical value with the mean of the target variable for that category. Useful when we want to incorporate target variable information into the encoding. It is effective for improving model performance especially in classification tasks.
Reply

You can use df.sample(5) for taking different data points from data.
Reply

Mahboob ul-Hassan
Assignment:
Assignment:
Types of feature encoding:
1- Ordinal Encoding
2- One-Hot Encoding
3- Binary Encoding
4- Label Encoding
5- Count Encoding
6- Target Encoding or Mean Encoding
7- Frequency Encoding
8- Feature Hashing
9- Embedding Layers
10-Entity Embeddings of Categorical Variables
A- Ordinal Encoding is used for categorical variables which have an inherent order or ranking
B- One-Hot Encoding is used for nominal categorical variables i.e. categories with no inherent order.
C- Binary Encoding is used with high-cardinality nominal categorical variables. D- Label Encoding is used when the ordinal relationship between categories is known and meaningful.
E- Count Encoding is used when frequency of occurrences of a category is relevant information.
F- Target Encoding /Mean Encoding is used when the relationship between the categorical variable and the target variable is important.
G- Frequency Encoding is used when the frequency of categories is relevant.
H-Feature Hashing is used when dealing with high-cardinality categorical features to reduce dimensionality.
J- Embedding Layers is used for embedding layers when working with categorical variables in neural networks.
K-Entity Embeddings of Categorical Variables seful in deep learning scenarios for learning dense representations of categorical variables.
Reply