Join the conversation

Done
Reply
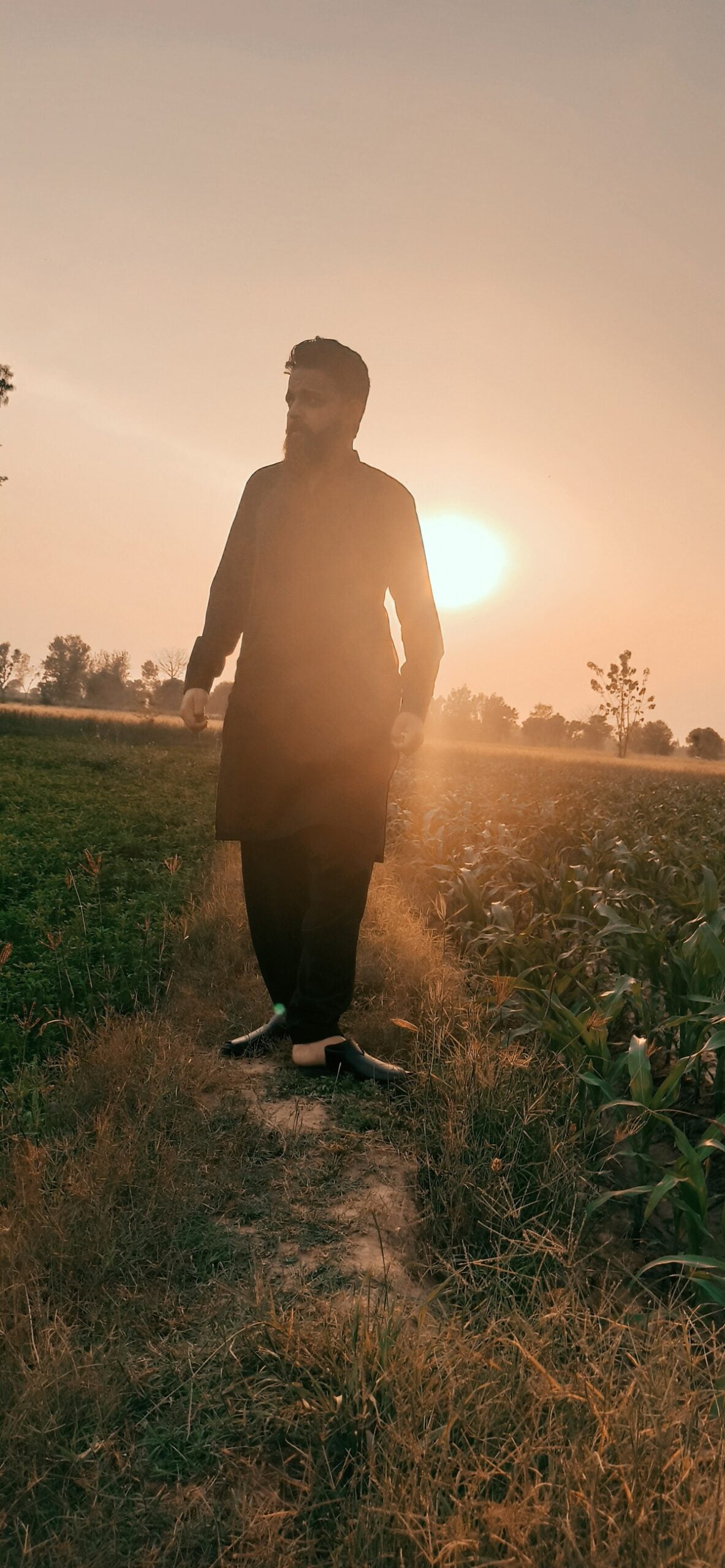
Done
Reply
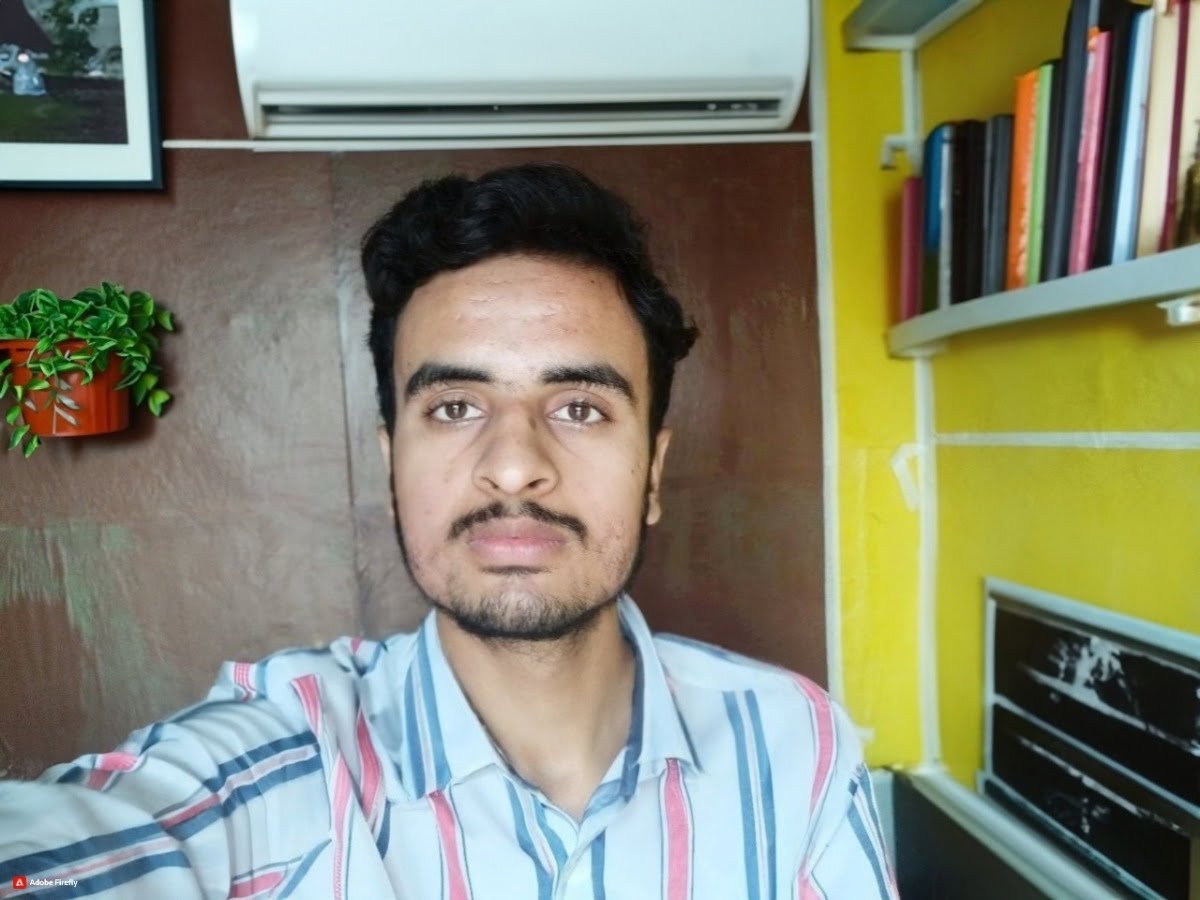
Done
Reply
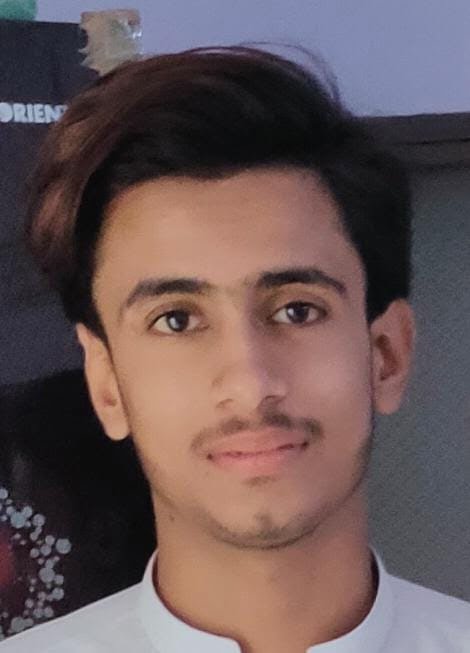
I learned in this video, Random Forest.
Reply
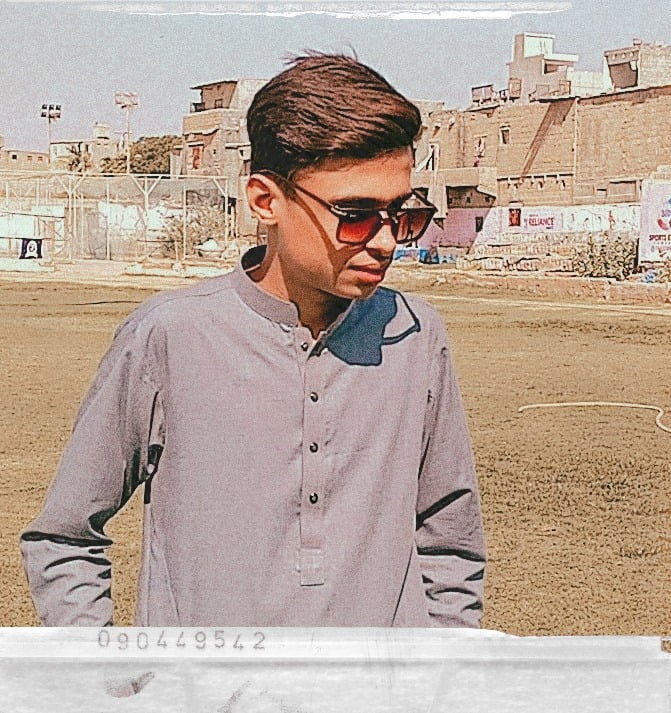
I learned in this lecture, Random Forest Theory.
Reply
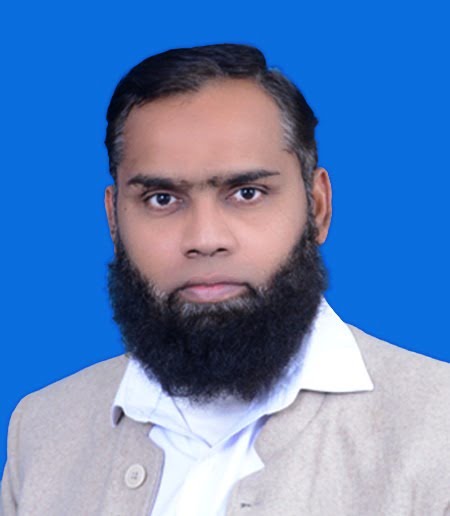
Random Forest algorithm comes in the Ensemble Method bagging type, which usually applies to large datasets. Further, I learned key features, advantages, applications, best practices, and challenges of the Random Forest algorithm.
Reply
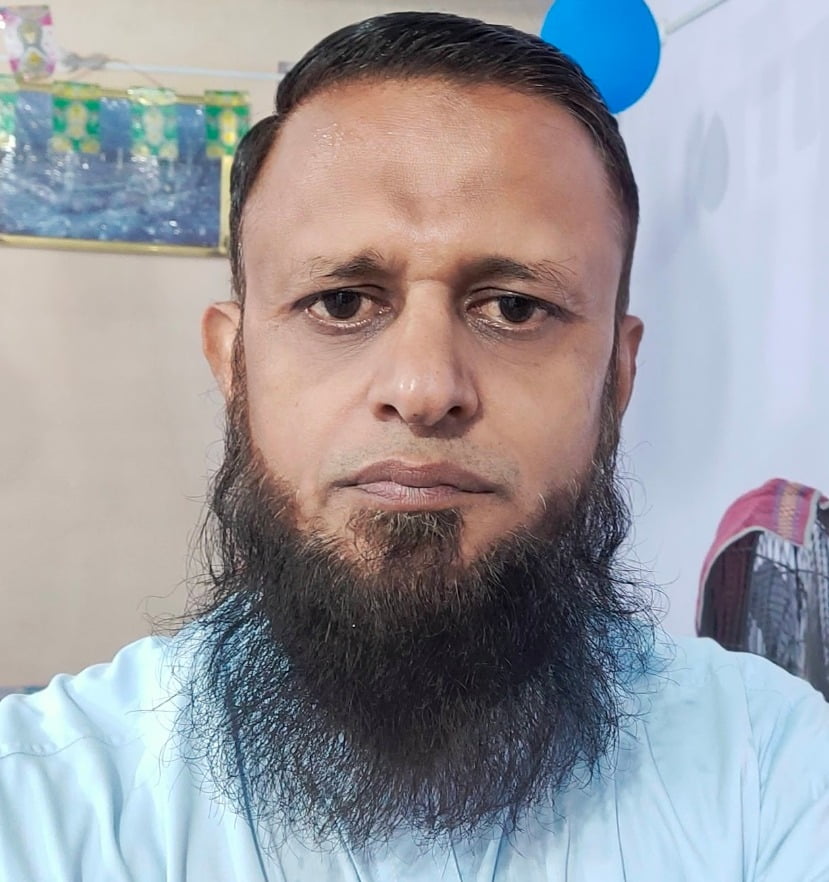
AOA, I learned in this lecture how random forests work.1-Bootstrap Aggregation (Bagging)
2-Random Feature Selection ( model more robust and prevents overfitting )
3-Aggregation of ResultsAnd I also learned key features of Random Forest, which are1-Robustness ( less prone to overfitting )
2-Handling Non-Linearity ( capable of handling non-linear data )
3-Feature Importance ( which feature is important to prediction )And I also learned best practices and considerations of RF, which are1-Numbers of tree ( more computational and cost-effective)
2-Depth of tree ( deeper trees can capture more information but might lead to overfitting )
3-Feature selection ( features are relevant and good quality )
ALLAH PAK aap ko sahat o aafiat wali lambi umar ata kray aor ap ko dono jahan ki bhalian naseeb farmaey,Ameen.
Reply