About Lesson
Notebook:
https://github.com/AammarTufail/six-months_python_for_data_science-mentorship-program/blob/main/07_machine_learning/16_boosting.ipynb
Join the conversation
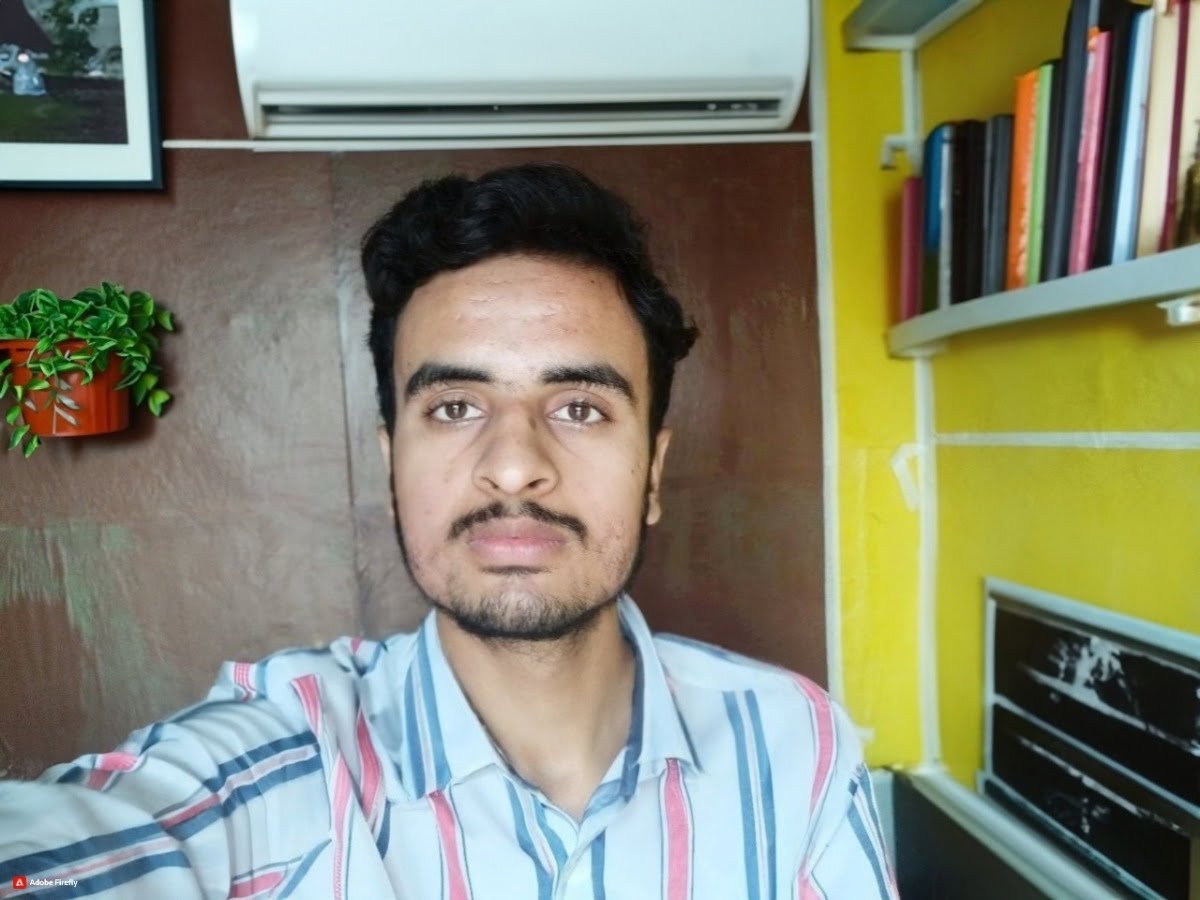
https://www.kaggle.com/muhammadrameez242 , ye meri kaggle ki I'd hai yha par Ap mukamal assignments simple roman urdu mein mill jai gi codes mein
Reply
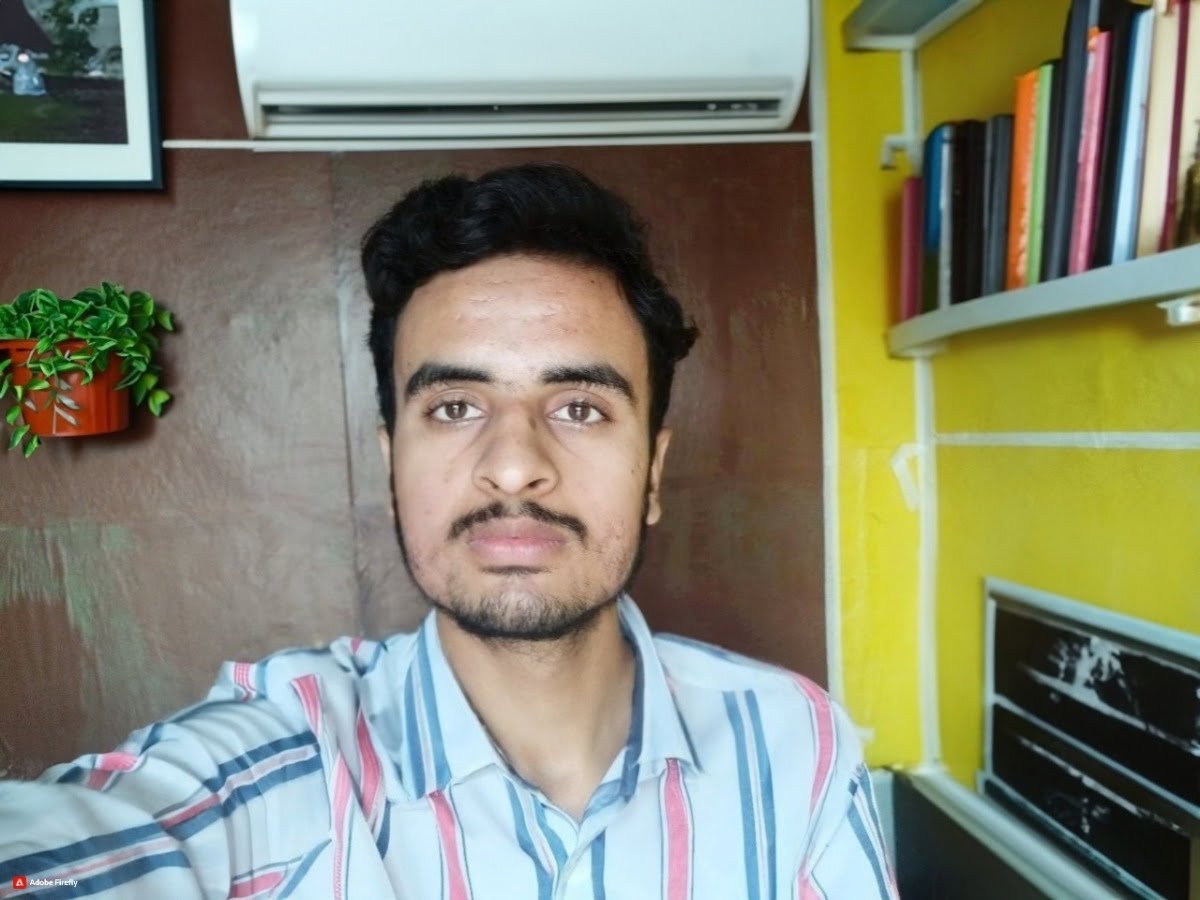
Done
Reply
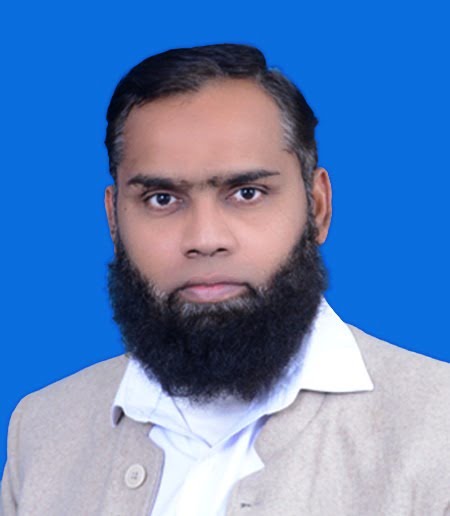
Boosting algorithms comparison in Python coding.
Reply
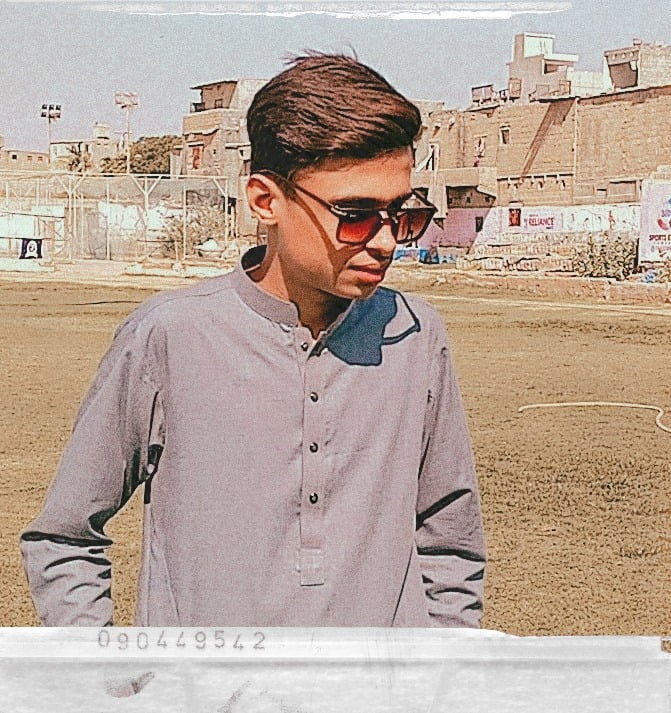
I have done this lecture with 100% practice.
Reply
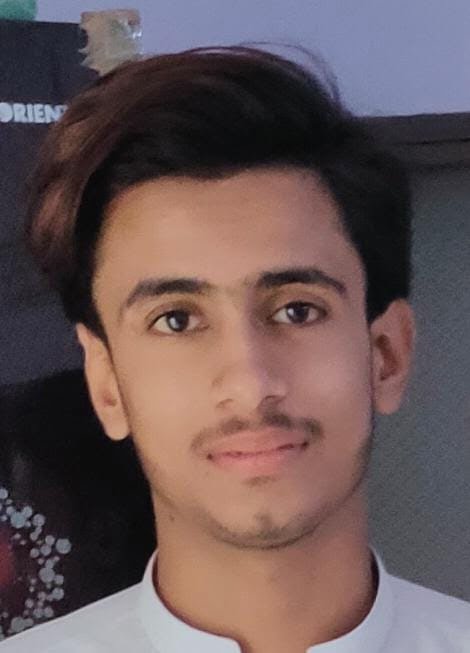
I have done this video with 100% practice.
Reply
AOA, shouldn't the prediction for each model be set in a different variable besides y_pred? y_pred is over written every time and has last xgboost model prediction values. Consequently bar plots are same for all three models. Thank you
Reply
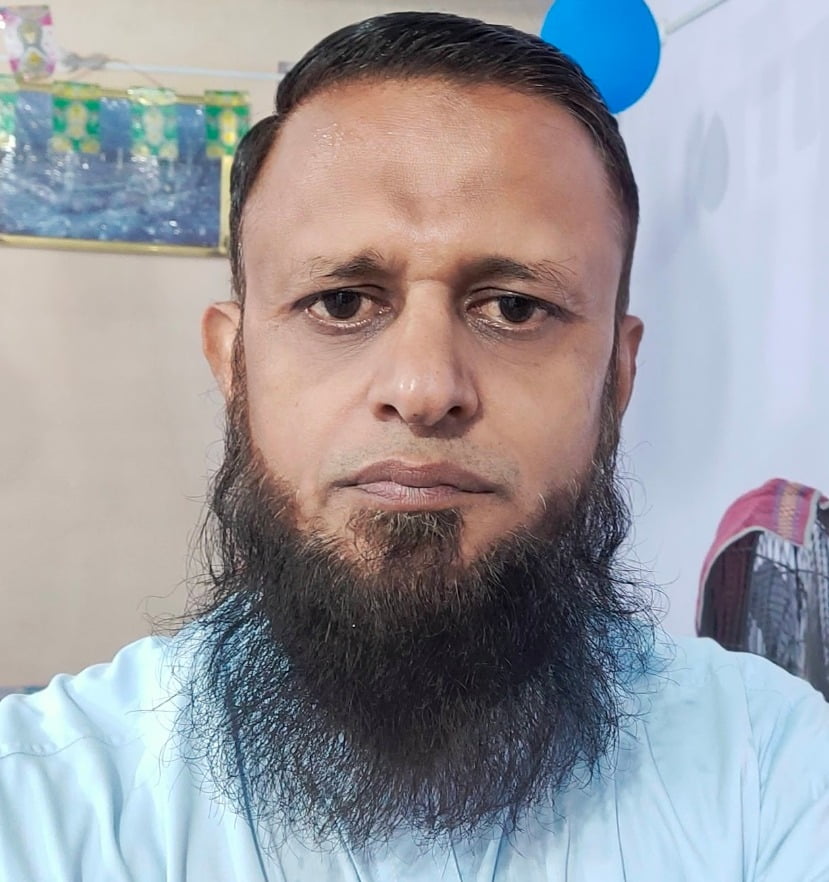
AOA, I also learned in this lecture how to code a boosting algorithm for classification in Python.Steps of Boosting in Python1-Import libraries
2-Import and load the dataset of DIMOND
3-Split the data into X and y.
4-Encode the input variables
5-Encode the target variable
6-Split the data into train and test sets
7-Train the decision tree model
8-Predict the test data
9-Train the random forest model
10-Predict the test data
11-Train the XGBoost model
12-Predict the test data
13-Make a bar plot showing each matrix with respect to the modelALLAH PAK aap ko sahat o aafiat wali lambi umar ata kray aor ap ko dono jahan ki bhalian naseeb farmaey or ap k walid-e-mohtram ko karwat karwat jannat ata farmay,Ameen.
Reply